Long-tail distribution image recognition method based on hierarchical learning
An image recognition and hierarchical technology, applied in the field of long-tail distribution image recognition based on hierarchical learning, can solve the problem of not improving the accuracy of tail category recognition.
- Summary
- Abstract
- Description
- Claims
- Application Information
AI Technical Summary
Problems solved by technology
Method used
Image
Examples
Embodiment Construction
[0060] The present invention will be further described below in conjunction with specific examples.
[0061] Taking the unbalanced ten class as an example, the method of the present invention is described in detail using the preprocessed CIFAR10 data set. For the original CIFAR10 dataset, there are 10 categories in total, the training set has 5000 pictures per category, and the test set has 1000 pictures per category. Select the first class in the training set to use all its samples, select the second class to sample 4600 samples without replacement, select the third class to sample 3000 samples without replacement, and so on. The smaller the number of samples, artificially construct a simple unbalanced long-tail distribution data set example, the example picture is as follows figure 1 shown.
[0062] Such as figure 2 As shown, the hierarchical learning-based long-tail distribution image recognition method provided in this embodiment includes the following steps:
[0063]...
PUM
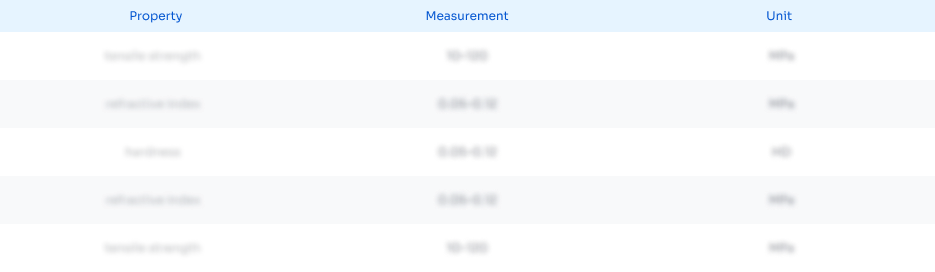
Abstract
Description
Claims
Application Information
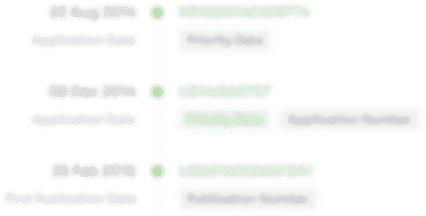
- R&D Engineer
- R&D Manager
- IP Professional
- Industry Leading Data Capabilities
- Powerful AI technology
- Patent DNA Extraction
Browse by: Latest US Patents, China's latest patents, Technical Efficacy Thesaurus, Application Domain, Technology Topic, Popular Technical Reports.
© 2024 PatSnap. All rights reserved.Legal|Privacy policy|Modern Slavery Act Transparency Statement|Sitemap|About US| Contact US: help@patsnap.com