AI calculation configuration method and device, equipment and storage medium
A configuration method and computing graph technology, applied in the field of deep learning, can solve problems affecting work efficiency, time-consuming and laborious, etc., and achieve the effect of improving efficiency, improving performance, and taking into account both computing speed and efficiency
- Summary
- Abstract
- Description
- Claims
- Application Information
AI Technical Summary
Problems solved by technology
Method used
Image
Examples
Embodiment 1
[0056] figure 1 The schematic flow chart of the AI computing configuration method provided in Embodiment 1 of the present invention is applicable to the separation of deep learning model computing graphs based on the data flow architecture. The method can be implemented by an AI computing configuration device, and can be realized by means of hardware or software . Such as figure 1 As shown, the AI computing configuration method provided by Embodiment 1 of the present invention includes:
[0057] S110. Obtain a computing graph based on the data flow architecture, where the computing graph includes multiple computing nodes.
[0058] Specifically, the calculation graph based on the data flow architecture refers to the calculation graph of the deep learning model developed based on the data flow architecture. Computational graph is a kind of computing process with directed acyclic graph as data structure, which includes multiple computing nodes, each computing node represen...
Embodiment 2
[0068] figure 2 It is a schematic flow chart of the AI computing configuration method provided by Embodiment 2 of the present invention, and this embodiment is a further optimization of the foregoing embodiment. Such as figure 2 As shown, the AI computing configuration method provided by Embodiment 2 of the present invention includes:
[0069] S210. Acquire a computing graph based on the data flow architecture, where the computing graph includes multiple computing nodes.
[0070] S220. Determine a node type of the computing node.
[0071] S230. Determine a corresponding executable device according to the node type.
[0072] S240. When there are multiple executable devices, determine an execution time required by each executable device to execute the computing node.
[0073] S250. Determine the execution condition of each executable device.
[0074] Specifically, the execution conditions of the executable device refer to the conditions under which the computing chip ...
Embodiment 3
[0084] Figure 3A It is a schematic flow chart of the AI computing configuration method provided by Embodiment 3 of the present invention, and this embodiment is a further optimization of the foregoing embodiments. Such as Figure 3A As shown, the AI computing configuration method provided by Embodiment 3 of the present invention includes:
[0085] S301. Acquire a computing graph based on a data flow architecture, where the computing graph includes multiple computing nodes.
[0086] S302. Determine a node type of the computing node.
[0087] S303. Determine a corresponding executable device according to the node type.
[0088] S304. When there are multiple executable devices, determine the execution time required by each executable device to execute the computing node.
[0089] S305. Determine the execution condition of each executable device.
[0090] S306. Determine the optimal execution device according to the execution conditions and execution time of each executabl...
PUM
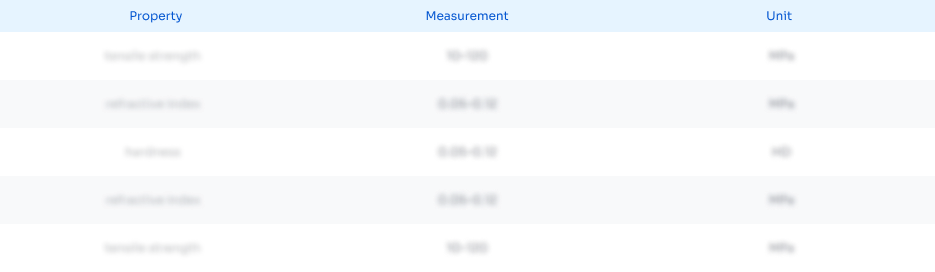
Abstract
Description
Claims
Application Information
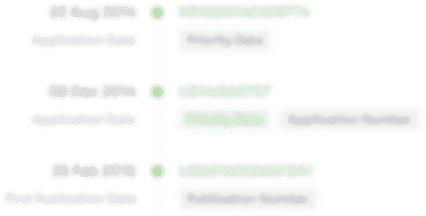
- R&D Engineer
- R&D Manager
- IP Professional
- Industry Leading Data Capabilities
- Powerful AI technology
- Patent DNA Extraction
Browse by: Latest US Patents, China's latest patents, Technical Efficacy Thesaurus, Application Domain, Technology Topic, Popular Technical Reports.
© 2024 PatSnap. All rights reserved.Legal|Privacy policy|Modern Slavery Act Transparency Statement|Sitemap|About US| Contact US: help@patsnap.com