Financial abnormity detection method and device based on multi-label learning
An anomaly detection and multi-label technology, applied in the computer field, can solve problems such as low accuracy, few data samples, and inability to detect financial whitewashing methods, and achieve the effects of improving efficiency, improving accuracy, and improving detection accuracy
- Summary
- Abstract
- Description
- Claims
- Application Information
AI Technical Summary
Benefits of technology
Problems solved by technology
Method used
Image
Examples
Embodiment Construction
[0047] The following will clearly and completely describe the technical solutions in the embodiments of the present invention with reference to the accompanying drawings in the embodiments of the present invention. Obviously, the described embodiments are only some, not all, embodiments of the present invention. Based on the embodiments of the present invention, all other embodiments obtained by persons of ordinary skill in the art without creative efforts fall within the protection scope of the present invention.
[0048] see figure 1 , is a schematic flowchart of an embodiment of the multi-label learning-based financial anomaly detection method provided by the present invention. Such as figure 1 The shown method includes step 101 to step 105, and each step is specifically as follows:
[0049] Step 101: Obtain the financial information of several enterprises, and generate eigenvector samples for each enterprise according to the financial information of each enterprise; wher...
PUM
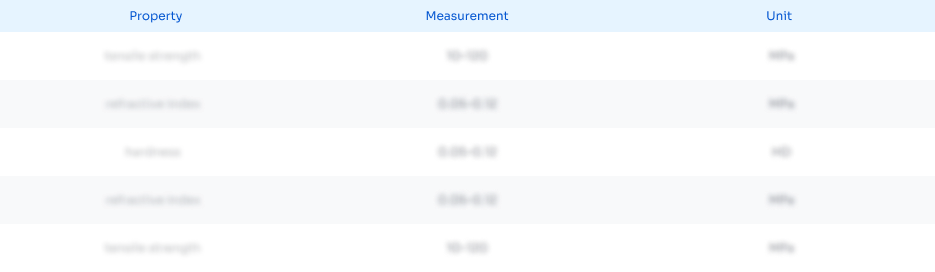
Abstract
Description
Claims
Application Information
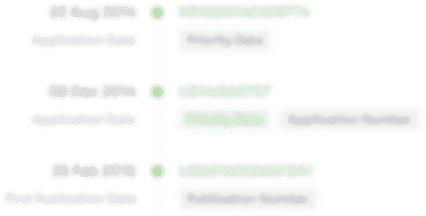
- R&D Engineer
- R&D Manager
- IP Professional
- Industry Leading Data Capabilities
- Powerful AI technology
- Patent DNA Extraction
Browse by: Latest US Patents, China's latest patents, Technical Efficacy Thesaurus, Application Domain, Technology Topic.
© 2024 PatSnap. All rights reserved.Legal|Privacy policy|Modern Slavery Act Transparency Statement|Sitemap