Method for classifying and segmenting sparse point clouds by utilizing point cloud density and rotation information
A point cloud density and sparse point technology, applied in the direction of instruments, character and pattern recognition, computer components, etc., can solve the problems of increasing data volume, calculation cost, and low calculation accuracy
- Summary
- Abstract
- Description
- Claims
- Application Information
AI Technical Summary
Problems solved by technology
Method used
Image
Examples
Embodiment Construction
[0066] The idea of this method closely revolves around the spatial structure correlation of rich and sparse point clouds, so as to use it to improve the accuracy and efficiency of classification and segmentation tasks. For the density and rotation information, this method designs a unique deep learning framework (step 3), which effectively enriches the spatial local correlation of sparse point clouds and greatly improves the performance of classification and segmentation, such as Figure 5 shown.
[0067] This method is divided into four steps, figure 2 It is an overview of the entire network process. Among them, step 2 is used to process the spatial coordinate information; step 3 is used to process the density and rotation information of the point cloud. Considering the variance of such information, an innovative deep learning network is designed in step 3 to provide such information, such as Figure 5 As shown, step 2 and step 3 are independent of each other, and the o...
PUM
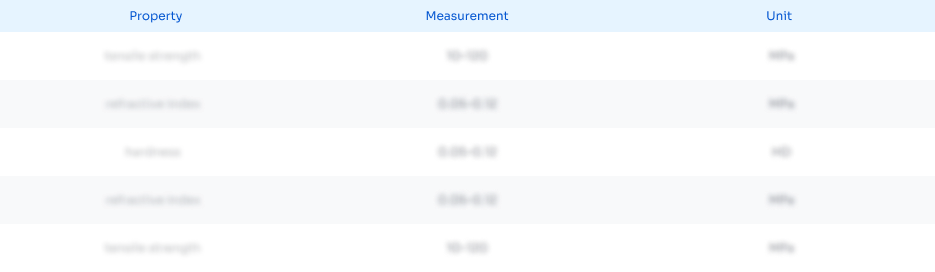
Abstract
Description
Claims
Application Information
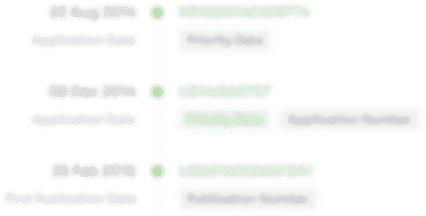
- R&D
- Intellectual Property
- Life Sciences
- Materials
- Tech Scout
- Unparalleled Data Quality
- Higher Quality Content
- 60% Fewer Hallucinations
Browse by: Latest US Patents, China's latest patents, Technical Efficacy Thesaurus, Application Domain, Technology Topic, Popular Technical Reports.
© 2025 PatSnap. All rights reserved.Legal|Privacy policy|Modern Slavery Act Transparency Statement|Sitemap|About US| Contact US: help@patsnap.com