Electroencephalogram signal denoising method based on width-depth echo state network
A technology of echo state network and EEG signal, applied in neural learning methods, biological neural network models, pattern recognition in signals, etc., can solve problems such as limited application range and inability to meet noise reduction performance
- Summary
- Abstract
- Description
- Claims
- Application Information
AI Technical Summary
Problems solved by technology
Method used
Image
Examples
Embodiment 1
[0070] Taking 10 EEG samples with a sampling frequency of 256 Hz and a sampling time of 30 minutes, each EEG sample has 460,800 sampling points and 23 electrode channels as an example, the signal denoising based on the width-depth echo state network of this embodiment The method consists of the following steps (see figure 1 , 2 ):
[0071] (1) Select EEG signal samples
[0072] Select s EEG signal samples from the Physionet database, and s in this embodiment is 10, as the output of the depth-width echo state network;
[0073] Each EEG signal sample is normalized according to the following formula:
[0074]
[0075] where x i is the sample data, where 1≤i≤10.
[0076] (2) Simulate noisy EEG signal samples
[0077] Adding noise with a signal-to-noise ratio of 0dB for the EEG signal sample is simulated into a noisy EEG signal sample. The noise in this embodiment adopts oculoelectric noise, and the noise-containing EEG sample is normalized according to formula (1). Use t...
Embodiment 2
[0125] Taking 10 EEG samples as an example, the sampling frequency is 256 Hz, the sampling time is 30 minutes, each EEG sample has 460,800 sampling points, and 23 electrode channels as an example, the signal denoising based on the width and depth echo state network in this embodiment The method consists of the following steps:
[0126] (1) Select EEG signal samples
[0127] This step is the same as in Example 1.
[0128] (2) Simulate noisy EEG signal samples
[0129] Add noise with a signal-to-noise ratio of -5dB to the EEG signal sample, and simulate it as a noisy EEG signal sample. Other steps in this step are the same as in Example 1.
[0130] (3) Divide network training set and test set
[0131] 70% of the EEG signal samples and noisy EEG samples were used as the network training set and 30% as the network test set by using the leave-out method, and there was no intersection between the test set and the training set.
[0132] (4) Build a network model
[0133] The wi...
Embodiment 3
[0161] Taking 10 EEG samples as an example, the sampling frequency is 256 Hz, the sampling time is 30 minutes, each EEG sample has 460,800 sampling points, and 23 electrode channels as an example, the signal denoising based on the width and depth echo state network in this embodiment The method consists of the following steps:
[0162] (1) Select EEG signal samples
[0163] This step is the same as in Example 1.
[0164] (2) Simulate noisy EEG signal samples
[0165] Add noise with a signal-to-noise ratio of 5dB to the EEG signal sample, and simulate it as a noisy EEG signal sample. Other steps in this step are the same as in Example 1.
[0166] (3) Divide network training set and test set
[0167] 90% of the EEG signal samples and noisy EEG samples are used as the network training set and 10% as the network test set by using the leave-out method, and there is no intersection between the test set and the training set.
[0168] (4) Build a network model
[0169] The width...
PUM
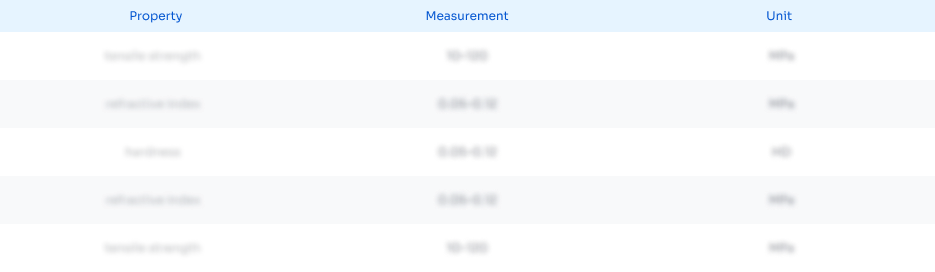
Abstract
Description
Claims
Application Information
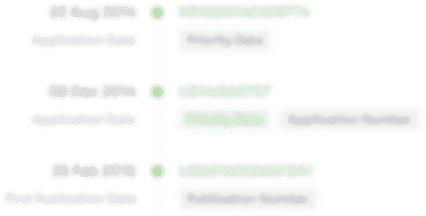
- R&D Engineer
- R&D Manager
- IP Professional
- Industry Leading Data Capabilities
- Powerful AI technology
- Patent DNA Extraction
Browse by: Latest US Patents, China's latest patents, Technical Efficacy Thesaurus, Application Domain, Technology Topic, Popular Technical Reports.
© 2024 PatSnap. All rights reserved.Legal|Privacy policy|Modern Slavery Act Transparency Statement|Sitemap|About US| Contact US: help@patsnap.com