IPv6 address discovery method and device based on gated convolutional variational auto-encoder
A self-encoder, ipv6 address technology, applied in the network field, to achieve the effect of improving sensitivity and improving model effect
- Summary
- Abstract
- Description
- Claims
- Application Information
AI Technical Summary
Problems solved by technology
Method used
Image
Examples
example 1
[0062] Example 1 Address Classification Experiment
[0063] The present invention has carried out experiments on address classification, and Table 1 shows the model effect after not using the address classification method, using manual classification and unsupervised clustering respectively. Experiments on three methods use the public dataset IPv6 Hitlist as a training seed set and scan to identify active targets.
[0064] In the experiment, the IPv6 Hitlist data set is used as the seed set for training, and after the training, the generator is used to generate candidate targets after 100,000 samples. This experiment removes duplicate candidate targets, and finally obtains effective generated address targets. Experiments show that the address classification method can indeed improve the generation effect of the model. Among them, the most generated addresses are Fixed IID of manual classification and Cluster 1 of unsupervised clustering. However, Low 64-bit subnet, SLAAC EU...
example 2
[0067] Example 2 model comparison experiment
[0068] This experiment compares 6GCVAE with the traditional variational autoencoder and the current cutting-edge address generation algorithm Entropy / IP. Table 2 shows the comparison results.
[0069] In order to verify the advantages of 6GCVAE, this experiment builds traditional variational autoencoder models by replacing the key component of 6GCVAE, the gated convolutional layer, and compares them with the present invention. After training the model with the IPv6 Hitlist dataset, the experiment generates 1,000,000 samples for comparison. Experiments show that the FNN VAE is difficult to complete the task of IPv6 object generation because the feed-forward neural network cannot capture semantic information well. RNN VAE and Convolutional VAE only focus on sequence relationship or structural information, so the number of generation is less. By promoting simple RNN layer to LSTM or GRU, the model outperforms RNN VAE. Finally, 6G...
PUM
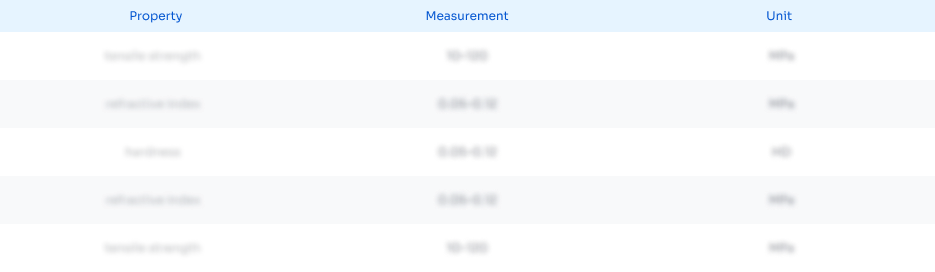
Abstract
Description
Claims
Application Information
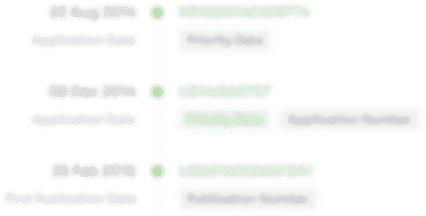
- R&D Engineer
- R&D Manager
- IP Professional
- Industry Leading Data Capabilities
- Powerful AI technology
- Patent DNA Extraction
Browse by: Latest US Patents, China's latest patents, Technical Efficacy Thesaurus, Application Domain, Technology Topic, Popular Technical Reports.
© 2024 PatSnap. All rights reserved.Legal|Privacy policy|Modern Slavery Act Transparency Statement|Sitemap|About US| Contact US: help@patsnap.com