Discrete supervision cross-modal hash retrieval method based on semantic preservation
A cross-modal, hashing technology, applied in the field of discrete-supervised cross-modal hash retrieval based on semantic preservation, can solve problems such as high computational complexity and memory overhead, difficult to solve, and loss of scalability.
- Summary
- Abstract
- Description
- Claims
- Application Information
AI Technical Summary
Problems solved by technology
Method used
Image
Examples
Embodiment
[0140] This embodiment takes the public dataset Mirflickr25K as an example, which contains 25,000 image and text sample pairs, and all sample pairs are distributed in 24 categories. First remove the labels that appear less than 20 times, and then remove the sample pairs that do not contain any labels and categories. There are 20015 sample pairs left in the data set. The image and text samples in the data set were extracted with CaffeNet and BOW (Bag Of Words) algorithms, respectively, with 4096-dimensional CNN features and 500-dimensional BOW features, and the features were averaged. 75% (15011) of the sample pairs are randomly selected to constitute the training set, while the remaining 25% (5004) of the sample pairs constitute the test set. In order to objectively evaluate the retrieval performance of the method of the present invention, the average accuracy rate MPA@r is used as the evaluation standard, where r represents the number of returned samples, and r=100 in the exp...
PUM
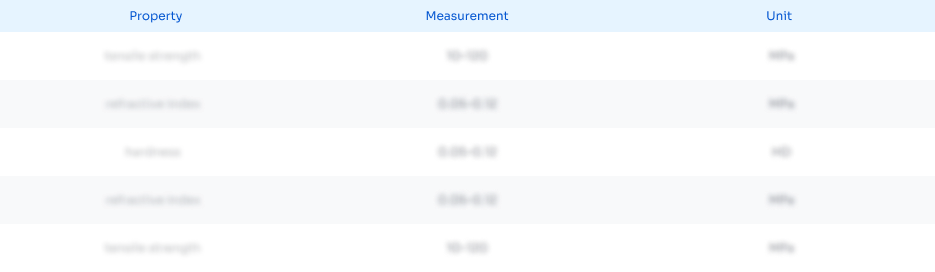
Abstract
Description
Claims
Application Information
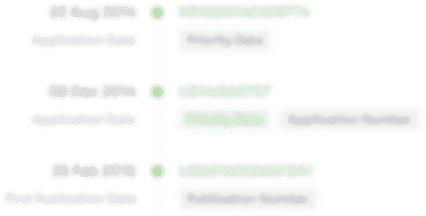
- R&D Engineer
- R&D Manager
- IP Professional
- Industry Leading Data Capabilities
- Powerful AI technology
- Patent DNA Extraction
Browse by: Latest US Patents, China's latest patents, Technical Efficacy Thesaurus, Application Domain, Technology Topic, Popular Technical Reports.
© 2024 PatSnap. All rights reserved.Legal|Privacy policy|Modern Slavery Act Transparency Statement|Sitemap|About US| Contact US: help@patsnap.com