Cluster electric vehicle charging behavior optimization method based on deep reinforcement learning
An electric vehicle and reinforcement learning technology, applied in electric vehicle charging technology, electric vehicles, charging stations, etc., can solve problems such as discrete action space, poor stability, and difficulty in training convergence
- Summary
- Abstract
- Description
- Claims
- Application Information
AI Technical Summary
Problems solved by technology
Method used
Image
Examples
Embodiment Construction
[0077]The present invention provides a charging behavior optimization method for cluster electric vehicles based on deep reinforcement learning, the optimization method is a charging optimization management method for cluster electric vehicles based on deep reinforcement learning; the optimization method is based on a double-delay deep deterministic strategy The gradient (twin delay DDPG, TD3) algorithm realizes the modeling of the continuously adjustable charging process of electric vehicles, trains the agent to control the charging power, optimizes the charging behavior of electric vehicles, and transfers the load when the time-of-use electricity price is high to when the electricity price is low Transfer to achieve the purpose of reducing user charging expenses and smoothing the peak load of the power grid; the charging process of a single electric vehicle is modeled through the twin delay deep deterministic policy gradient algorithm (twin delay deep deterministic policy grad...
PUM
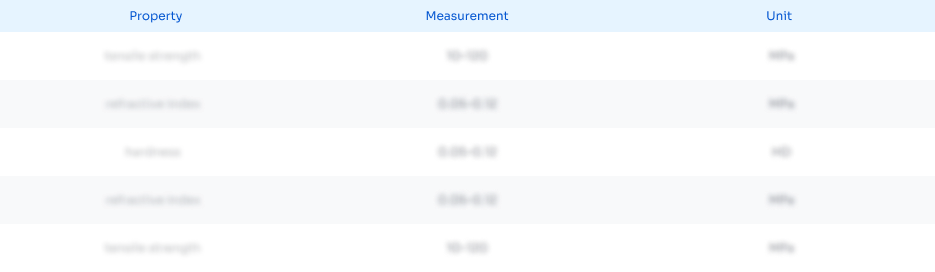
Abstract
Description
Claims
Application Information
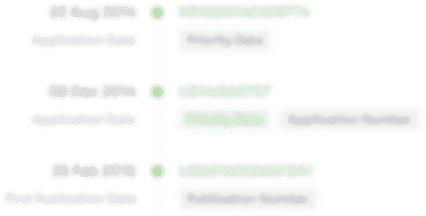
- R&D Engineer
- R&D Manager
- IP Professional
- Industry Leading Data Capabilities
- Powerful AI technology
- Patent DNA Extraction
Browse by: Latest US Patents, China's latest patents, Technical Efficacy Thesaurus, Application Domain, Technology Topic.
© 2024 PatSnap. All rights reserved.Legal|Privacy policy|Modern Slavery Act Transparency Statement|Sitemap