Reinforcement learning strategy learning method based on potential action representation space
A strategy learning and representation space technology, applied in the field of machine learning, can solve problems such as costing a lot of time and money, mechanical damage, damage to intelligent systems, etc., to achieve the effect of improving generalization ability, stability, and learning speed
- Summary
- Abstract
- Description
- Claims
- Application Information
AI Technical Summary
Problems solved by technology
Method used
Image
Examples
Embodiment Construction
[0020] The following will clearly and completely describe the technical solutions in the embodiments of the present invention with reference to the accompanying drawings in the embodiments of the present invention. Obviously, the described embodiments are only some of the embodiments of the present invention, not all of them. Based on the embodiments of the present invention, all other embodiments obtained by persons of ordinary skill in the art without making creative efforts belong to the protection scope of the present invention.
[0021] The embodiment of the present invention discloses a reinforcement learning strategy learning method based on latent action representation space, which specifically includes: model modeling, optimization target construction, and optimization problem solving. In the implementation of the present invention, the interaction process between the agent and the environment is modeled as a Markov decision process (MDP), which can be represented by a...
PUM
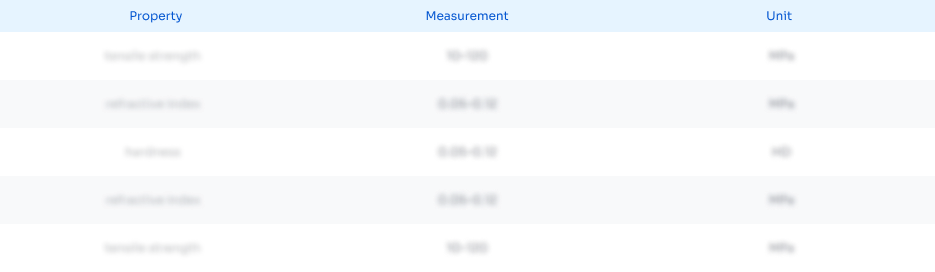
Abstract
Description
Claims
Application Information
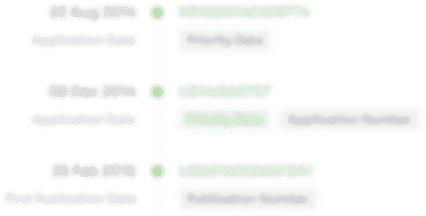
- R&D Engineer
- R&D Manager
- IP Professional
- Industry Leading Data Capabilities
- Powerful AI technology
- Patent DNA Extraction
Browse by: Latest US Patents, China's latest patents, Technical Efficacy Thesaurus, Application Domain, Technology Topic, Popular Technical Reports.
© 2024 PatSnap. All rights reserved.Legal|Privacy policy|Modern Slavery Act Transparency Statement|Sitemap|About US| Contact US: help@patsnap.com