Remote sensing image small target detection method based on feedback type multi-scale training
A small target detection and remote sensing image technology, applied in image data processing, neural learning methods, graphics and image conversion, etc., can solve the problems of unsatisfactory small target detection performance and poor model performance in remote sensing images, etc. The effect of fitting phenomenon and category imbalance phenomenon, improving accuracy and enhancing detection ability
- Summary
- Abstract
- Description
- Claims
- Application Information
AI Technical Summary
Problems solved by technology
Method used
Image
Examples
Embodiment Construction
[0042] The specific implementation manner and working principle of the present invention will be further described in detail below in conjunction with the accompanying drawings.
[0043] Such as figure 1 As shown, a small target detection method for remote sensing images based on feedback multi-scale training, the specific steps are as follows:
[0044] Step 1. Build a feedback multi-scale convolutional neural network composed of a detection module and a feedback multi-scale training module, such as figure 2 As shown, use the pre-trained model to initialize the network weights, and input the original image data to train it in an end-to-end manner, specifically:
[0045] The construction steps of the detection module are as follows:
[0046] Step 11, build as image 3 The feature extraction network shown is used to extract the high-level semantic features and low-level semantic features of the input image, and then normalize the size through upsampling, and obtain the added...
PUM
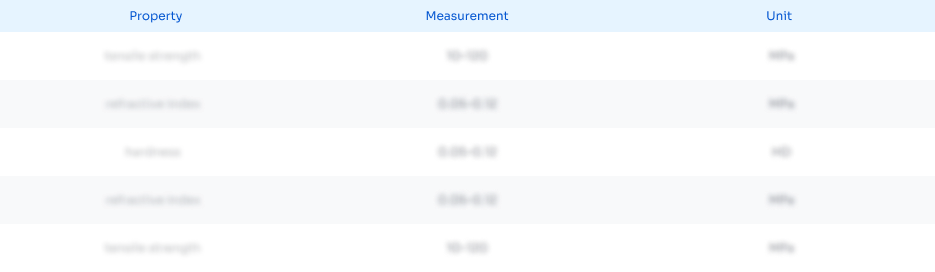
Abstract
Description
Claims
Application Information
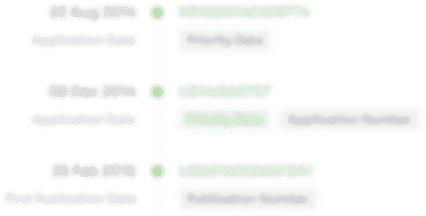
- R&D Engineer
- R&D Manager
- IP Professional
- Industry Leading Data Capabilities
- Powerful AI technology
- Patent DNA Extraction
Browse by: Latest US Patents, China's latest patents, Technical Efficacy Thesaurus, Application Domain, Technology Topic.
© 2024 PatSnap. All rights reserved.Legal|Privacy policy|Modern Slavery Act Transparency Statement|Sitemap