Image description optimization method based on pointer network
A technology for image description and optimization methods, applied in neural learning methods, biological neural network models, instruments, etc., can solve problems such as general accuracy, large model structure, and high training overhead
- Summary
- Abstract
- Description
- Claims
- Application Information
AI Technical Summary
Problems solved by technology
Method used
Image
Examples
Embodiment
[0114] The present invention can be used for picture record summaries in daily life. Any picture taken by the user can be generated with the help of the model trained by the present invention. Viewing and browsing in the future is also in line with people's needs for fast retrieval and classification of pictures in the era of big data.
[0115] In order to verify the effectiveness of the present invention, the present invention was trained and tested on the Microsoft COCO 2014 data set. The data set has a total of 123,287 pictures, and each picture has 4 to 5 artificially provided annotation sentences. According to the division principle of Karpathy Split, 113287 pictures are used as training pictures (train set), 5000 pictures are used for verification (val set), and 5000 pictures are used for testing (test set). The present invention utilizes a GTX 1080Ti graphics card to accelerate training, adopts an Adam learner, and sets the learning rate to 2e-4.
[0116] Some paramete...
PUM
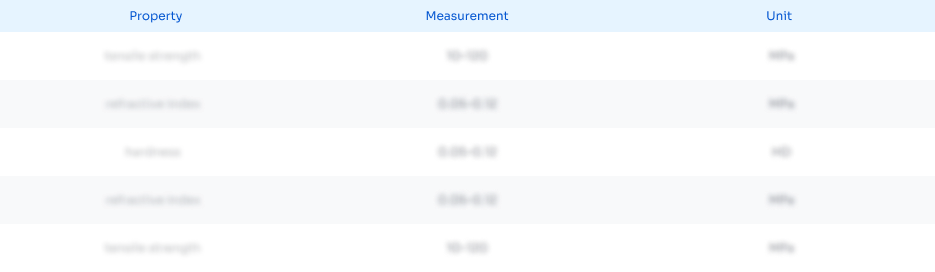
Abstract
Description
Claims
Application Information
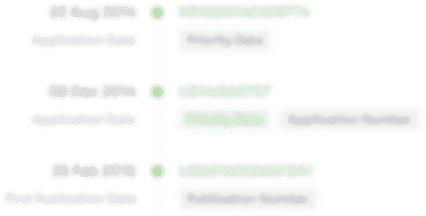
- R&D
- Intellectual Property
- Life Sciences
- Materials
- Tech Scout
- Unparalleled Data Quality
- Higher Quality Content
- 60% Fewer Hallucinations
Browse by: Latest US Patents, China's latest patents, Technical Efficacy Thesaurus, Application Domain, Technology Topic, Popular Technical Reports.
© 2025 PatSnap. All rights reserved.Legal|Privacy policy|Modern Slavery Act Transparency Statement|Sitemap|About US| Contact US: help@patsnap.com