Vertical Domain Entity Disambiguation Method Fused with Topic Model and Convolutional Neural Network
A convolutional neural network and topic model technology, applied in biological neural network models, neural architecture, character and pattern recognition, etc., can solve the problems of referential error, unable to consider lexical and word order, difficult to reflect contextual semantic influence and constraints, etc. , to improve the accuracy, optimize the complexity of text processing, and improve the accuracy of disambiguation
- Summary
- Abstract
- Description
- Claims
- Application Information
AI Technical Summary
Problems solved by technology
Method used
Image
Examples
Embodiment Construction
[0047] The present invention will be described in further detail below in conjunction with the accompanying drawings.
[0048]In order to effectively overcome the disadvantages of traditional disambiguation methods, the present invention adopts a multi-model fusion method in order to effectively extract text features and improve the accuracy of disambiguation results. At present, the word vector model in natural language processing maps each word to a high-latitude vector through corpus training, which can contain more information than the bag of words model. The LDA topic model extracts the features of the local information of the context, and the topic features obtained have a clearer topic direction than the global one, which can effectively disambiguate the domain. In recent years, the convolutional neural network model, which has been widely used, has achieved great success in the field of natural language processing. The multi-layer convolution operation can effectively ...
PUM
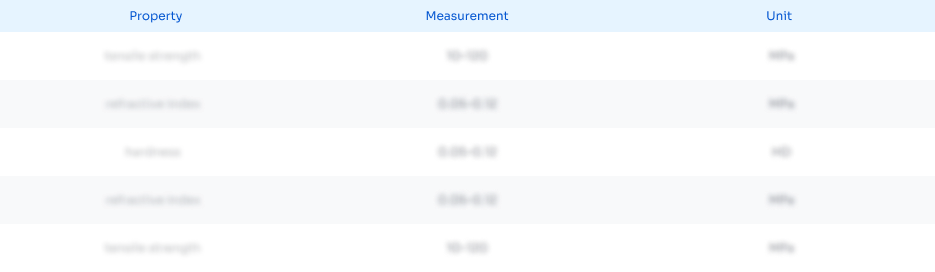
Abstract
Description
Claims
Application Information
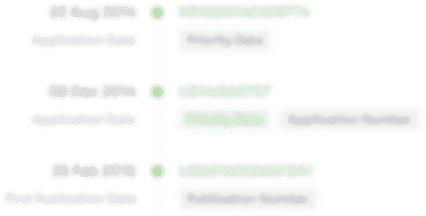
- R&D Engineer
- R&D Manager
- IP Professional
- Industry Leading Data Capabilities
- Powerful AI technology
- Patent DNA Extraction
Browse by: Latest US Patents, China's latest patents, Technical Efficacy Thesaurus, Application Domain, Technology Topic.
© 2024 PatSnap. All rights reserved.Legal|Privacy policy|Modern Slavery Act Transparency Statement|Sitemap