Graph neutral networks with attention
A neural network and attention technology, applied in the field of graph neural network with attention, can solve the problems of high computational complexity, high cost of verification or verification, and exacerbation
- Summary
- Abstract
- Description
- Claims
- Application Information
AI Technical Summary
Problems solved by technology
Method used
Image
Examples
Embodiment Construction
[0078] Embodiments of the present invention are described below by way of example only. These examples represent the best mode currently known to applicants for putting the invention into practice, although they are not the only ways of carrying out the invention. Describes the functionality of the example and the sequence of steps for constructing and operating the example. However, the same or equivalent functions and sequences can be implemented by different examples.
[0079] The present invention relates to a system, apparatus and method for efficiently generating and training a robust graph neural network (GNN) model using attention weights and based on data representing at least a portion of an entity-entity graph dataset, the entity - Entity graph datasets are for example, but not limited to, generated from datasets of facts and / or entity relationships / associations, etc.; GNN models can be used for predictive tasks, e.g., for example, but not limited to, Link predict...
PUM
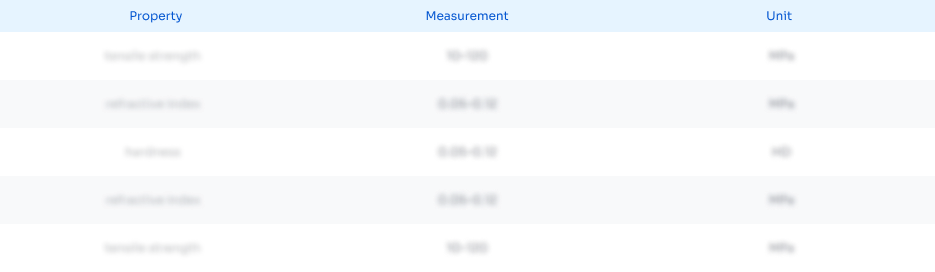
Abstract
Description
Claims
Application Information
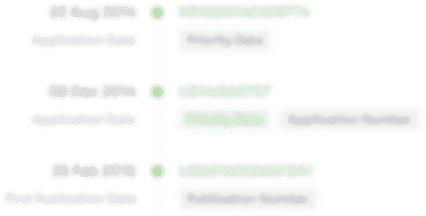
- R&D Engineer
- R&D Manager
- IP Professional
- Industry Leading Data Capabilities
- Powerful AI technology
- Patent DNA Extraction
Browse by: Latest US Patents, China's latest patents, Technical Efficacy Thesaurus, Application Domain, Technology Topic, Popular Technical Reports.
© 2024 PatSnap. All rights reserved.Legal|Privacy policy|Modern Slavery Act Transparency Statement|Sitemap|About US| Contact US: help@patsnap.com