Image classification method based on integrated knowledge distillation
A classification method and knowledge technology, applied in the field of image classification based on integrated knowledge distillation, can solve problems such as performance gaps, and achieve the effects of good classification accuracy, soft output, and simple training process
- Summary
- Abstract
- Description
- Claims
- Application Information
AI Technical Summary
Problems solved by technology
Method used
Image
Examples
Embodiment Construction
[0028] The present invention will be further described in detail below with reference to the accompanying drawings and embodiments. It should be noted that the following embodiments are intended to facilitate the understanding of the present invention, but do not limit it in any way.
[0029] Such as figure 1 As shown, an image classification method based on integrated knowledge distillation includes the following steps:
[0030] S01, pre-trained teacher model
[0031] This embodiment uses the ImageNet-2012 data set as the image classification training data set. The ImageNet-2012 dataset contains 1.28 million training images, with a total of 1000 categories.
[0032] Before training, image transformation processing is performed on each picture. For details, please refer to "Deepresidual learning for image recognition" published on IEEE Conference on Computer Vision and Pattern Recognition, the top conference on computer vision. The stochastic gradient descent algorithm is u...
PUM
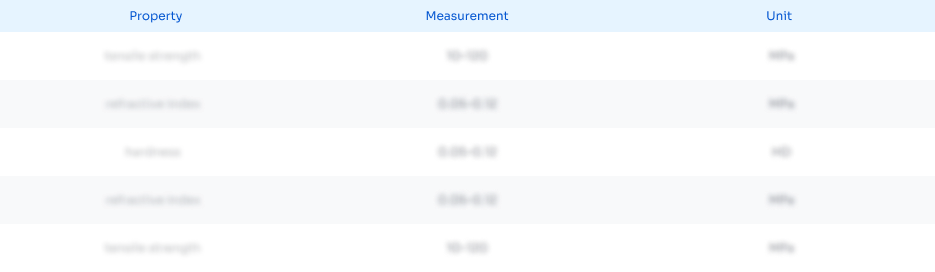
Abstract
Description
Claims
Application Information
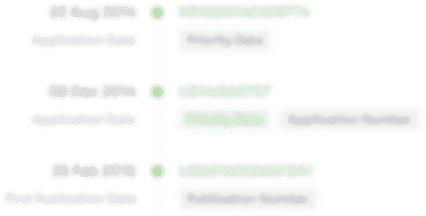
- R&D Engineer
- R&D Manager
- IP Professional
- Industry Leading Data Capabilities
- Powerful AI technology
- Patent DNA Extraction
Browse by: Latest US Patents, China's latest patents, Technical Efficacy Thesaurus, Application Domain, Technology Topic, Popular Technical Reports.
© 2024 PatSnap. All rights reserved.Legal|Privacy policy|Modern Slavery Act Transparency Statement|Sitemap|About US| Contact US: help@patsnap.com