A Deep Generative Adversarial Method for Denoising Underwater Acoustic Signals
An underwater acoustic signal and depth technology, applied in the recognition of patterns in signals, neural learning methods, biological neural network models, etc., to achieve the effect of strong self-applicability, elimination of strong dependencies and over-fitting problems
- Summary
- Abstract
- Description
- Claims
- Application Information
AI Technical Summary
Problems solved by technology
Method used
Image
Examples
Embodiment Construction
[0037] Step 1 First, the samples are divided into frames and processed in batches.
[0038] Step 2 Then send the processed data into the generative model for model training. The generative model is a semi-supervised model, so there is a difference between the trained data and the clean data
[0039] Step 3: Add the data generated by the generator to the noisy data, and send it to the discriminant model together with the original clean and noisy data for discrimination. The discriminator can discriminate well at the beginning. The data of the generated model is a fake sample, and the output is 0. The original clean and noisy data is the real sample and the output is 1. According to the result of the discriminator, the generator starts to simulate its own generated data, so that the data is as close to the real data as possible, so that until the discriminator has no way to distinguish, the generator will generate The data is sent to the discriminator again, and the discriminato...
PUM
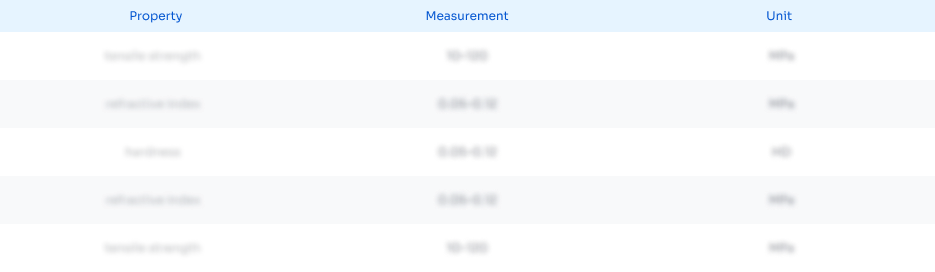
Abstract
Description
Claims
Application Information
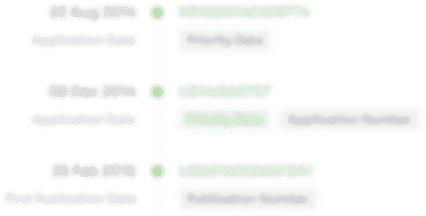
- R&D
- Intellectual Property
- Life Sciences
- Materials
- Tech Scout
- Unparalleled Data Quality
- Higher Quality Content
- 60% Fewer Hallucinations
Browse by: Latest US Patents, China's latest patents, Technical Efficacy Thesaurus, Application Domain, Technology Topic, Popular Technical Reports.
© 2025 PatSnap. All rights reserved.Legal|Privacy policy|Modern Slavery Act Transparency Statement|Sitemap|About US| Contact US: help@patsnap.com