Hyperspectral target detection method based on L1 regular constraint depth multi-example learning
A multi-instance learning and target detection technology, applied in the field of image processing, can solve the problems of large memory space, long solution process, and long time-consuming SVM classifier, so as to enhance generalization ability, avoid over-fitting, and improve detection effect Effect
- Summary
- Abstract
- Description
- Claims
- Application Information
AI Technical Summary
Problems solved by technology
Method used
Image
Examples
Embodiment Construction
[0037] The embodiments and effects of the present invention will be further described in detail below in conjunction with the accompanying drawings.
[0038] refer to figure 1 , the implementation steps of the present invention are as follows:
[0039] Step 1. Construct multi-instance learning data.
[0040] (1.1) Input a hyperspectral image, and use 80% of the images as a training set, and the remaining 20% of the images as a test set;
[0041] The data used in this example includes a total of 5 hyperspectral images, of which the first, second, third and fourth images are used as training sets, and the fifth image is used as a test set;
[0042] (1.2) Define each pixel in the hyperspectral image as an example, K examples form a package, divide the training set images into N packages, and define the package containing the target example as a positive package, and the package that does not contain the target example A bag is defined as a negative bag;
[0043] In this exa...
PUM
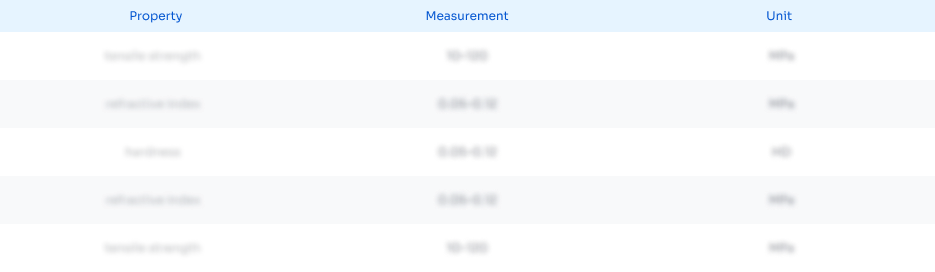
Abstract
Description
Claims
Application Information
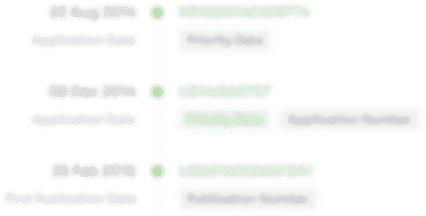
- R&D Engineer
- R&D Manager
- IP Professional
- Industry Leading Data Capabilities
- Powerful AI technology
- Patent DNA Extraction
Browse by: Latest US Patents, China's latest patents, Technical Efficacy Thesaurus, Application Domain, Technology Topic, Popular Technical Reports.
© 2024 PatSnap. All rights reserved.Legal|Privacy policy|Modern Slavery Act Transparency Statement|Sitemap|About US| Contact US: help@patsnap.com