Fraud node identification method based on graph embedded representation and recurrent neural network
A technology of cyclic neural network and embedded representation, which is applied in the field of network security, can solve problems such as large network data volume and complex semi-structured data structure, and achieve the effect of reducing labor costs and reducing dimensions
- Summary
- Abstract
- Description
- Claims
- Application Information
AI Technical Summary
Problems solved by technology
Method used
Image
Examples
Embodiment
[0058] In order to explain the purpose, technical solutions and main points of the present invention more clearly and in detail, the present invention will be further elaborated in detail. It should be understood that the implementation methods described here are only used to explain the specific methods of the present invention, rather than limit the present invention , those skilled in the art can implement and popularize according to the principles described in the present invention, and simply modify the user relationship network datasets that need to be processed, so that the present invention can be extended to similar application scenarios.
[0059]The present invention first preprocesses the original data, then uses the preprocessed data to train the graph neural network, and finally the trained neural network predicts the target label according to the test data, specifically including the preprocessing stage, the training model stage and the use of The three stages of ...
PUM
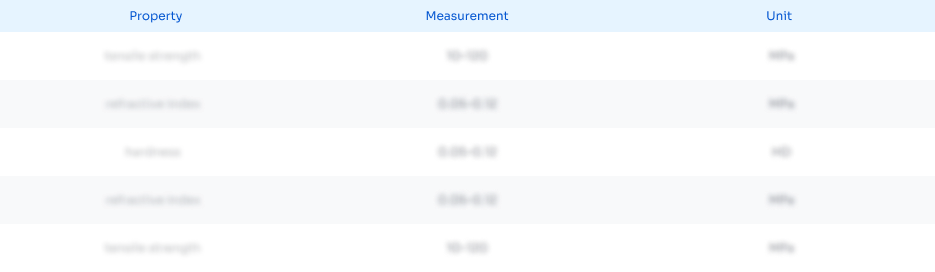
Abstract
Description
Claims
Application Information
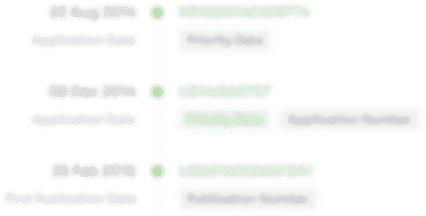
- Generate Ideas
- Intellectual Property
- Life Sciences
- Materials
- Tech Scout
- Unparalleled Data Quality
- Higher Quality Content
- 60% Fewer Hallucinations
Browse by: Latest US Patents, China's latest patents, Technical Efficacy Thesaurus, Application Domain, Technology Topic, Popular Technical Reports.
© 2025 PatSnap. All rights reserved.Legal|Privacy policy|Modern Slavery Act Transparency Statement|Sitemap|About US| Contact US: help@patsnap.com