Lightweight remote sensing target detection method based on SE-YOLOv3
A target detection, lightweight technology, applied in the field of computer vision and deep learning, can solve problems such as unsatisfactory results, low recall rate, and dense target distribution
- Summary
- Abstract
- Description
- Claims
- Application Information
AI Technical Summary
Problems solved by technology
Method used
Image
Examples
Embodiment Construction
[0044] The technical solutions in the embodiments of the present invention will be described clearly and in detail below with reference to the drawings in the embodiments of the present invention. The described embodiments are only some of the embodiments of the invention.
[0045] The technical scheme that the present invention solves the problems of the technologies described above is:
[0046] The embodiment of the present invention is based on the YOLOv3 target detection framework as the basic framework. For details, see Redmon J, FarhadiA. Yolov3: An incremental improvement [J]. arXiv preprint arXiv: 1804.02767, 2018. Among them, the backbone network of the network is modified to a lightweight structure, which is composed of depth-separable convolutions. The extracted features are output with uniform scale features through the SPP module, and then strengthened by the attention module SE as the input of the next layer of the network.
[0047] Below in conjunction with acc...
PUM
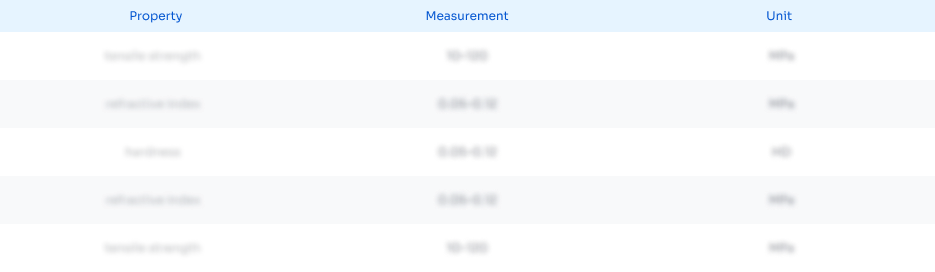
Abstract
Description
Claims
Application Information
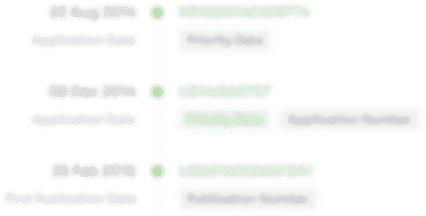
- R&D Engineer
- R&D Manager
- IP Professional
- Industry Leading Data Capabilities
- Powerful AI technology
- Patent DNA Extraction
Browse by: Latest US Patents, China's latest patents, Technical Efficacy Thesaurus, Application Domain, Technology Topic, Popular Technical Reports.
© 2024 PatSnap. All rights reserved.Legal|Privacy policy|Modern Slavery Act Transparency Statement|Sitemap|About US| Contact US: help@patsnap.com