Method for enhancing CT image quality and resolution based on deep learning
A CT image and deep learning technology, applied in the field of image processing, can solve the problems of image detail loss, image denoising, etc., and achieve the effect of improving the quality of CT images
- Summary
- Abstract
- Description
- Claims
- Application Information
AI Technical Summary
Problems solved by technology
Method used
Image
Examples
Embodiment 1
[0024] Such as figure 1 As shown, the present embodiment proposes a method for enhancing CT image quality and resolution based on deep learning, which mainly includes the following steps:
[0025] S1. Preprocessing the collected clinical data to obtain a data set.
[0026] S2. Construct a deep learning model including a generator network, a decision network and a perception network.
[0027] S3. Constructing a loss function.
[0028] S4. Utilize the data set and the loss function to update the parameters of the iterative generation network to obtain a trained deep learning model.
[0029] S5. Input the low-quality and low-resolution images into the trained deep learning model to obtain high-quality and high-resolution images.
[0030] Specifically, the process of preprocessing clinical data in step S1 includes the following:
[0031] S11. Acquire low-quality CT images with low radiation dose and low resolution and high-quality CT images with normal radiation dose and high ...
Embodiment 2
[0055] The difference from Example 1 is that in this example, the collected clinical data are preprocessed to obtain the data set. The low-quality CT image is obtained by three-dimensional interpolation method to obtain the same size as the high-quality CT image, and then obtained after cropping. Pairs of small chunks of data.
[0056] The generation network can be but not limited to U-Net structure, and the decision device can be but not limited to Patch GAN. The use of Patch GAN can take into account the influence of different parts of the image, and solve the problem of inconsistent output images caused by only one corresponding output for one input. precise question.
[0057] Unlike the simulation training set used in the prior art, the present invention obtains a real training data set by preprocessing real clinical data, so that the deep learning model can be applied to clinical practice. The deep learning model of the present invention can optimize low-radiation and lo...
PUM
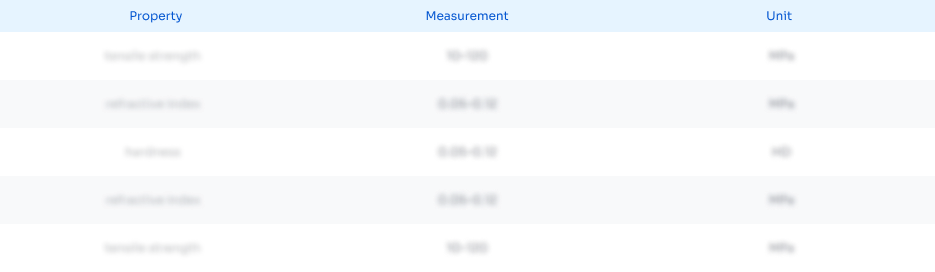
Abstract
Description
Claims
Application Information
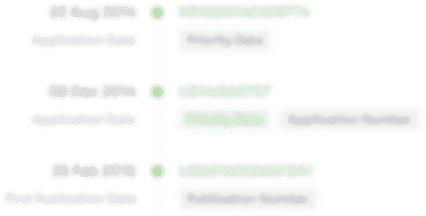
- Generate Ideas
- Intellectual Property
- Life Sciences
- Materials
- Tech Scout
- Unparalleled Data Quality
- Higher Quality Content
- 60% Fewer Hallucinations
Browse by: Latest US Patents, China's latest patents, Technical Efficacy Thesaurus, Application Domain, Technology Topic, Popular Technical Reports.
© 2025 PatSnap. All rights reserved.Legal|Privacy policy|Modern Slavery Act Transparency Statement|Sitemap|About US| Contact US: help@patsnap.com