Assembly and method for predicting the remaining service life of a machine
A machine and life-span technology, applied in reasoning methods, neural learning methods, instruments, etc., to achieve the effect of strong predictive power
- Summary
- Abstract
- Description
- Claims
- Application Information
AI Technical Summary
Problems solved by technology
Method used
Image
Examples
Embodiment Construction
[0021] figure 1 A flowchart for predicting the remaining useful life of a machine is shown. First, the sensor detects sensor data of the operation of the machine. Sensor measurements of vibrations are of particular importance in many cases. A machine is for example an engine in a production facility. The sensor data are collected decentralized in the peripheral units and forwarded to the memory-programmable control unit.
[0022] For monitoring the engine, for example two to three sensors are placed, such as vibration sensors and acceleration sensors, which can be supplemented, if necessary, with temperature sensors and strain gauges. The sensor is preferably arranged on the drive side on the bearing shield, as close as possible to the bearing or the shaft. The measuring direction of the vibration sensor is preferably oriented transversely to the axis.
[0023] exist figure 1 A state detection module, not shown in more detail, derives state data A from the sensor data, f...
PUM
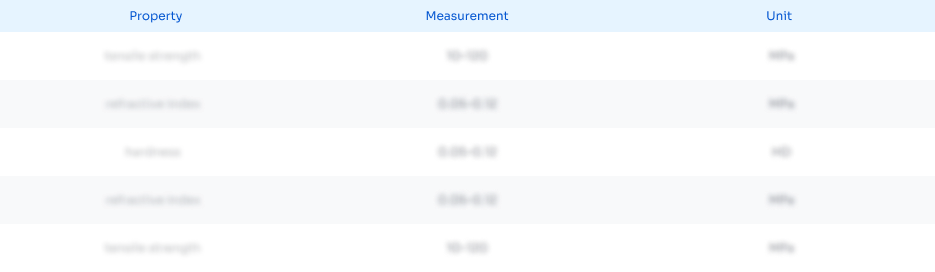
Abstract
Description
Claims
Application Information
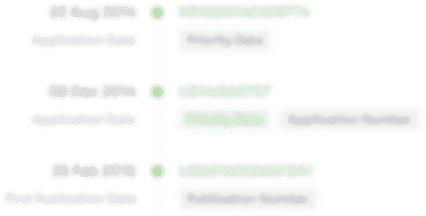
- R&D
- Intellectual Property
- Life Sciences
- Materials
- Tech Scout
- Unparalleled Data Quality
- Higher Quality Content
- 60% Fewer Hallucinations
Browse by: Latest US Patents, China's latest patents, Technical Efficacy Thesaurus, Application Domain, Technology Topic, Popular Technical Reports.
© 2025 PatSnap. All rights reserved.Legal|Privacy policy|Modern Slavery Act Transparency Statement|Sitemap|About US| Contact US: help@patsnap.com