Method for improving overall classification accuracy of long-tail distribution speech based on transfer learning
A transfer learning and accuracy technology, applied in the field of deep learning network training, can solve the problems of insufficient representation ability, unbalanced data distribution, insufficient amount of tail category data, etc., to achieve the effect of improving the effect.
- Summary
- Abstract
- Description
- Claims
- Application Information
AI Technical Summary
Problems solved by technology
Method used
Image
Examples
Embodiment
[0017] Feature extraction is performed on the original speech data in the long-tail distribution speech data set to obtain the logarithmic Mel features corresponding to the speech data.
[0018] figure 1 The upper part shows the fitting of the CNN network to the logarithmic Mel feature: the obtained logarithmic Mel feature is processed as the input of the CNN network to extract the speech features, and the parameter θ of the network model is obtained after training n =(w n ,b n ), where n represents the number of network layers of CNN, w is the weight value, b is the offset, and θ represents the parameters of this layer; the data distribution used in the first model training presents a long-tail distribution; Processing: that is, use the features obtained above to perform timing modeling and analyze the timing information of the features;
[0019] figure 1 The lower part shows the migration learning process, through the first model training, the model parameters θ of the C...
PUM
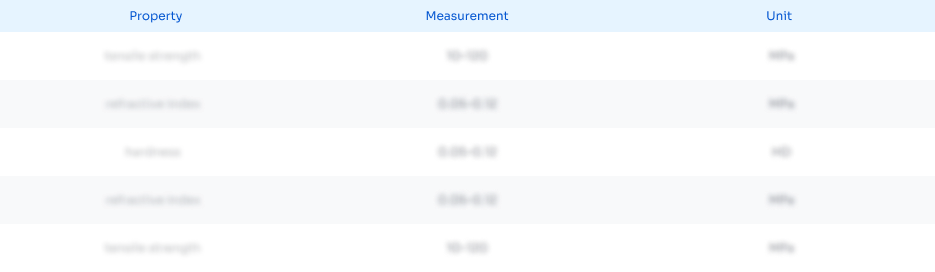
Abstract
Description
Claims
Application Information
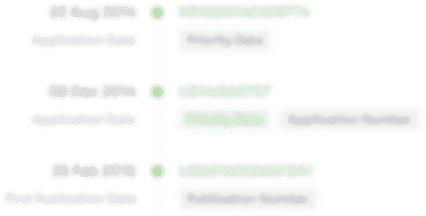
- R&D Engineer
- R&D Manager
- IP Professional
- Industry Leading Data Capabilities
- Powerful AI technology
- Patent DNA Extraction
Browse by: Latest US Patents, China's latest patents, Technical Efficacy Thesaurus, Application Domain, Technology Topic, Popular Technical Reports.
© 2024 PatSnap. All rights reserved.Legal|Privacy policy|Modern Slavery Act Transparency Statement|Sitemap|About US| Contact US: help@patsnap.com