Low-resolution ship classification method of double-flow feature learning generative adversarial network
A low-resolution, high-resolution technique for low-resolution ship classification with two-stream feature learning Generative Adversarial Networks
- Summary
- Abstract
- Description
- Claims
- Application Information
AI Technical Summary
Problems solved by technology
Method used
Image
Examples
Embodiment Construction
[0048] like figure 1 As shown in the figure, a low-resolution ship classification method of a dual-stream feature learning generative adversarial network of the present invention includes the following steps:
[0049] Step 1. Establish a ship image training set: select multiple high-resolution ship images of different types of ships in the HRSC data set to form a high-resolution ship image set, and the number of images in the high-resolution ship image set is not less than 500 , perform low-resolution processing on each high-resolution ship image in the high-resolution ship image set to obtain a low-resolution ship image corresponding to each high-resolution ship image, multiple high-resolution ship images and their corresponding low-resolution ship images rate ship images to form a ship image training set;
[0050] Each high-resolution ship image in the ship image training set and its corresponding low-resolution ship image form a ship image training group;
[0051] In this...
PUM
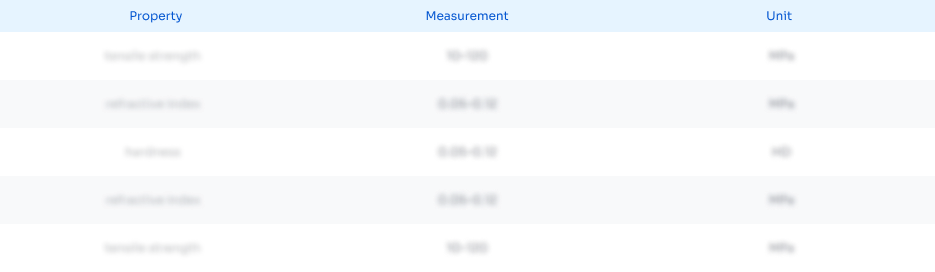
Abstract
Description
Claims
Application Information
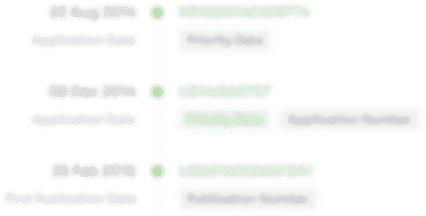
- R&D Engineer
- R&D Manager
- IP Professional
- Industry Leading Data Capabilities
- Powerful AI technology
- Patent DNA Extraction
Browse by: Latest US Patents, China's latest patents, Technical Efficacy Thesaurus, Application Domain, Technology Topic, Popular Technical Reports.
© 2024 PatSnap. All rights reserved.Legal|Privacy policy|Modern Slavery Act Transparency Statement|Sitemap|About US| Contact US: help@patsnap.com