Lightweight image classification method, system and device based on model pruning
A classification method and lightweight technology, applied in the field of computer vision and deep learning, can solve the problems of unmeasured features and classification task correlation, and achieve the effect of reducing the loss of image classification accuracy, good feature representation ability, and easy deployment and application
- Summary
- Abstract
- Description
- Claims
- Application Information
AI Technical Summary
Problems solved by technology
Method used
Image
Examples
example
[0074] This example is tested on the standard dataset CIFAR-10. CIFAR-10 is an image classification data set. All images are three-channel color images with a size of 32×32, containing 60,000 pictures, of which 50,000 are for the training set and 10,000 are for the verification set. The deep neural network used in the experiment in this example is AlexNet, VGG16 and residual network ResNet.
[0075] In order to clearly see the changes before and after compression, this example first uses different convolutional neural networks to train a full-precision 32-bit model. In the compression process, the pre-trained 32-bit model is used as the initialization of the quantized model. In the process of pre-training and pruning, the data pre-processing uses the method of data enhancement, filling 0 on the original 32*32 image boundary and expanding it to a 36*36 image, and then randomly cropping it into a 32*32 image, and then Randomly flip left and right.
[0076] Table 1 Optimizatio...
PUM
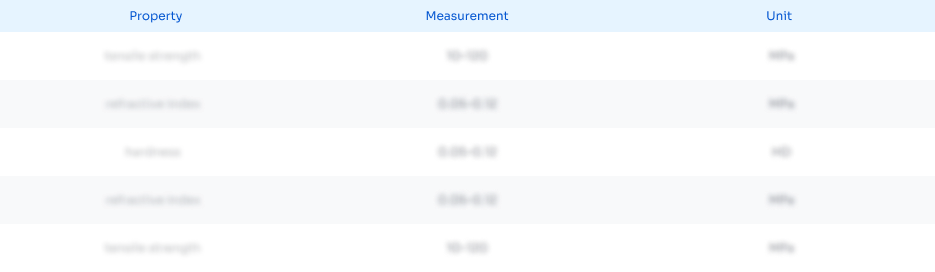
Abstract
Description
Claims
Application Information
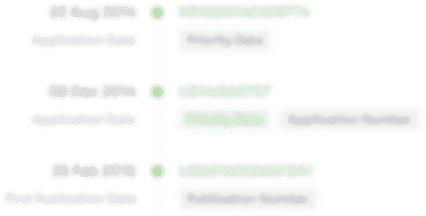
- R&D Engineer
- R&D Manager
- IP Professional
- Industry Leading Data Capabilities
- Powerful AI technology
- Patent DNA Extraction
Browse by: Latest US Patents, China's latest patents, Technical Efficacy Thesaurus, Application Domain, Technology Topic, Popular Technical Reports.
© 2024 PatSnap. All rights reserved.Legal|Privacy policy|Modern Slavery Act Transparency Statement|Sitemap|About US| Contact US: help@patsnap.com