Electronic laryngoscope image classification method based on binuclear convolution feature extraction
A feature extraction and electronic throat technology, applied in the field of computer vision and pattern recognition, can solve the problems of low efficiency and accuracy of classification and recognition
- Summary
- Abstract
- Description
- Claims
- Application Information
AI Technical Summary
Problems solved by technology
Method used
Image
Examples
Embodiment Construction
[0036] The present invention will be further described below in conjunction with the accompanying drawings. The following examples are only used to illustrate the technical solution of the present invention more clearly, but not to limit the protection scope of the present invention.
[0037] Step 1: Data preprocessing stage, such as figure 1 shown;
[0038] Step 2: First, we extract a picture currently to be processed from step 1, such as figure 2 , entered into image 3 In the dual-kernel convolutional feature extraction network. Secondly, in order to demonstrate the effect of network extraction features, we obtain the features of the previous layer of the fully connected layer and visualize them, as follows: Figure 4 result. Finally, the image gets a 1*512-dimensional image feature F through the fully connected layer.
[0039] F=[0,0,0.24433999,0,0.7739287,2.735432,1.2492355,0,0,5.9589076,...,2.735432,1.2492355,0,0,0,5.9589076]
[0040] Step 3: Input the F obtained i...
PUM
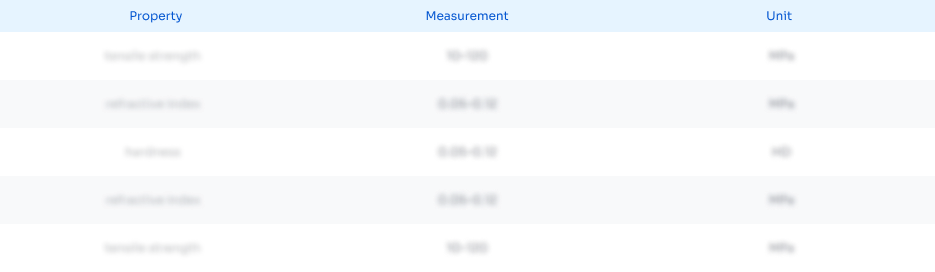
Abstract
Description
Claims
Application Information
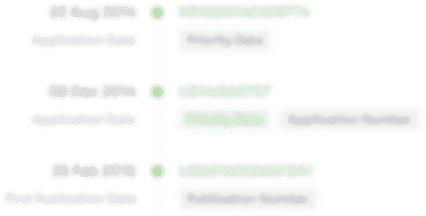
- R&D Engineer
- R&D Manager
- IP Professional
- Industry Leading Data Capabilities
- Powerful AI technology
- Patent DNA Extraction
Browse by: Latest US Patents, China's latest patents, Technical Efficacy Thesaurus, Application Domain, Technology Topic, Popular Technical Reports.
© 2024 PatSnap. All rights reserved.Legal|Privacy policy|Modern Slavery Act Transparency Statement|Sitemap|About US| Contact US: help@patsnap.com