Lung lobe segmentation method based on 3D full convolutional neural network and multi-task learning
A convolutional neural network and multi-task learning technology, which is applied in the field of lung lobe segmentation based on 3D full convolutional neural network and multi-task learning, can solve the problem of inaccurate results, difficult results of lung lobe segmentation by the network, and large amount of neural network parameters, etc. problem, to achieve the effect of accurate lung lobe segmentation
- Summary
- Abstract
- Description
- Claims
- Application Information
AI Technical Summary
Problems solved by technology
Method used
Image
Examples
Embodiment
[0042] The embodiment of the present invention proposes a lung lobe segmentation method based on 3D full convolutional neural network and multi-task learning, the flow chart of which is shown in figure 1 , wherein the method includes the following steps:
[0043] a. Prepare lung lobe-related data and perform calibration;
[0044] b. Preprocess the original lung lobe CT image data and accurately labeled lung lobe related data to remove redundant background information;
[0045] c. Construct a 3D fully convolutional neural network based on multi-task learning;
[0046] d, using preprocessed calibrated lobe-related data and synthetic learning errors to train the constructed 3D fully convolutional neural network;
[0047] e. Use the trained 3D fully convolutional neural network to perform lung lobe segmentation on the input 3D lung lobe CT image, and output the predicted lung lobe label.
[0048] On the basis of the above steps, in the implementation process of this embodiment,...
PUM
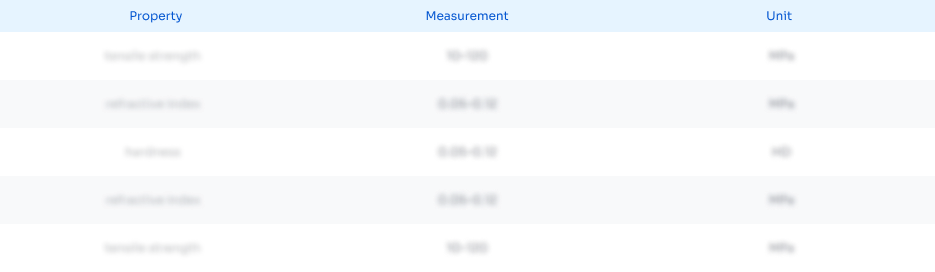
Abstract
Description
Claims
Application Information
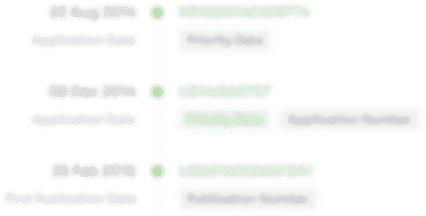
- Generate Ideas
- Intellectual Property
- Life Sciences
- Materials
- Tech Scout
- Unparalleled Data Quality
- Higher Quality Content
- 60% Fewer Hallucinations
Browse by: Latest US Patents, China's latest patents, Technical Efficacy Thesaurus, Application Domain, Technology Topic, Popular Technical Reports.
© 2025 PatSnap. All rights reserved.Legal|Privacy policy|Modern Slavery Act Transparency Statement|Sitemap|About US| Contact US: help@patsnap.com