Method for predicting residual life of rotating machinery under multiple working conditions based on dynamic domain adaptation network
A rotating machinery and dynamic technology, applied in the field of machinery, can solve the problems of low prediction accuracy and poor generalization ability of remaining life prediction of rotating machinery, and achieve the effect of improving model prediction accuracy and generalization ability.
- Summary
- Abstract
- Description
- Claims
- Application Information
AI Technical Summary
Problems solved by technology
Method used
Image
Examples
Embodiment Construction
[0058] The present invention will be described in further detail below in conjunction with the accompanying drawings and specific embodiments.
[0059] refer to figure 1 , the implementation steps of the present invention are further described in detail.
[0060] Step 1, construct a multi-layer convolutional neural network.
[0061] Build a 19-layer convolutional neural network consisting of four sub-modules with the same structure connected in series and then connected to the fully connected layer and the output layer. The structure of each sub-module consists of a convolutional layer, an activation function layer, a normalization layer and Pooling layer composition.
[0062] Set the parameters of each layer in the sub-module as follows: set the number of convolution kernels of the convolution layer in the first to fourth sub-modules to 4, 8, 16, and 32 respectively, and set the size of the convolution kernel to 3×1. The product step size is set to 2; the activation functi...
PUM
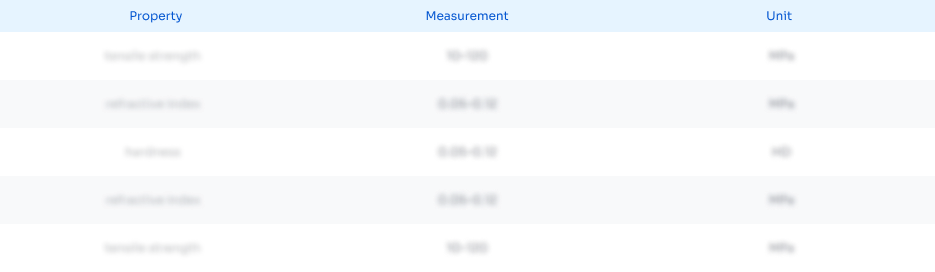
Abstract
Description
Claims
Application Information
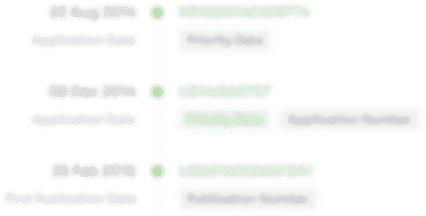
- R&D Engineer
- R&D Manager
- IP Professional
- Industry Leading Data Capabilities
- Powerful AI technology
- Patent DNA Extraction
Browse by: Latest US Patents, China's latest patents, Technical Efficacy Thesaurus, Application Domain, Technology Topic, Popular Technical Reports.
© 2024 PatSnap. All rights reserved.Legal|Privacy policy|Modern Slavery Act Transparency Statement|Sitemap|About US| Contact US: help@patsnap.com