Image classification network compression method based on convolution kernel of any shape
A technology of arbitrary shape, classification network, applied in image coding, image data processing, biological neural network model and other directions, can solve the problem of inability to remove redundant parameters of convolution kernel, irregular sparseness of network structure, inability to achieve, etc. Good network compression effect, high compression rate, and the effect of improving performance
- Summary
- Abstract
- Description
- Claims
- Application Information
AI Technical Summary
Problems solved by technology
Method used
Image
Examples
Embodiment Construction
[0026] Now in conjunction with embodiment, accompanying drawing, the present invention will be further described:
[0027] The present invention proposes an image classification network compression method based on an arbitrary shape convolution kernel, and its usage flow is as follows figure 1 As shown, the convolution calculation process of the arbitrary shape convolution kernel is as follows figure 2 shown. The specific embodiment of the present invention is described below in conjunction with image classification example, but technical content of the present invention is not limited to described scope, and specific embodiment comprises the following steps:
[0028] (1) Construct an image data set with a large number of training samples and labels, refer to classic networks such as VGG and ResNet to build a convolutional neural network for image classification, and modify the output number of the last fully connected layer of the network to the number of image categories ...
PUM
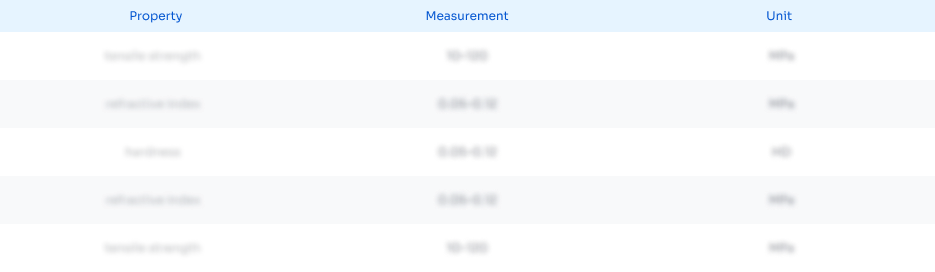
Abstract
Description
Claims
Application Information
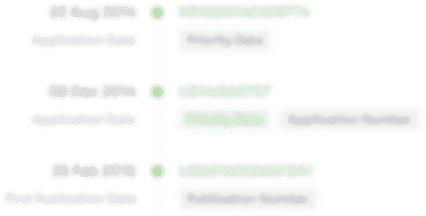
- R&D Engineer
- R&D Manager
- IP Professional
- Industry Leading Data Capabilities
- Powerful AI technology
- Patent DNA Extraction
Browse by: Latest US Patents, China's latest patents, Technical Efficacy Thesaurus, Application Domain, Technology Topic, Popular Technical Reports.
© 2024 PatSnap. All rights reserved.Legal|Privacy policy|Modern Slavery Act Transparency Statement|Sitemap|About US| Contact US: help@patsnap.com