Image classification network compression method based on convolution kernel shape automatic learning
A technology for automatic learning and classification of networks, applied in neural learning methods, biological neural network models, instruments, etc., can solve the problem of inability to remove redundant parameters of convolution kernels, achieve good network compression effect, improve performance, and reduce the amount of calculation. Effect
- Summary
- Abstract
- Description
- Claims
- Application Information
AI Technical Summary
Problems solved by technology
Method used
Image
Examples
Embodiment Construction
[0028] Now in conjunction with embodiment, accompanying drawing, the present invention will be further described:
[0029] The present invention proposes an image classification network compression method based on automatic learning of the shape of the convolution kernel. The automatic learning process of the shape of the convolution kernel is as follows: image 3 shown. The specific embodiment of the present invention is described below in conjunction with image classification example, but technical content of the present invention is not limited to described scope, and specific embodiment comprises the following steps:
[0030] (1) Build a convolutional neural network for image classification, and build an image dataset with a large number of training samples and labels.
[0031] (2) For the convolution layer in the neural network, the convolution calculation process of the conventional convolution kernel is:
[0032] Y=X*w
[0033] In the formula, is the input feature ...
PUM
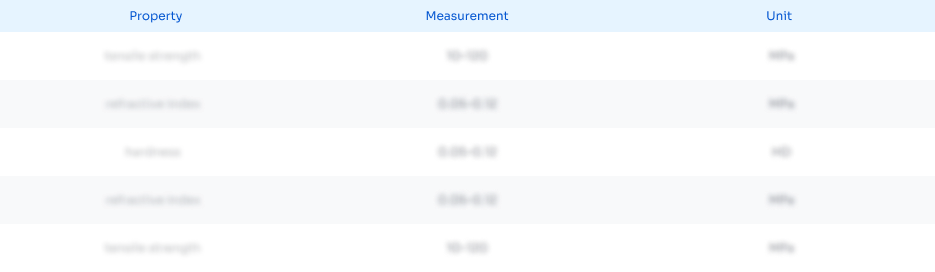
Abstract
Description
Claims
Application Information
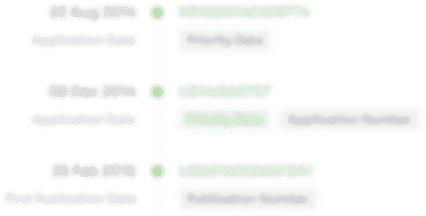
- R&D
- Intellectual Property
- Life Sciences
- Materials
- Tech Scout
- Unparalleled Data Quality
- Higher Quality Content
- 60% Fewer Hallucinations
Browse by: Latest US Patents, China's latest patents, Technical Efficacy Thesaurus, Application Domain, Technology Topic, Popular Technical Reports.
© 2025 PatSnap. All rights reserved.Legal|Privacy policy|Modern Slavery Act Transparency Statement|Sitemap|About US| Contact US: help@patsnap.com