Semantic segmentation method based on multi-source heterogeneous remote sensing image
A remote sensing image and semantic segmentation technology, applied in the field of deep learning, can solve problems such as large differences, decreased accuracy, and strict model design requirements.
- Summary
- Abstract
- Description
- Claims
- Application Information
AI Technical Summary
Problems solved by technology
Method used
Image
Examples
Embodiment Construction
[0044] The present invention will be further described below in conjunction with the accompanying drawings and embodiments.
[0045] The invention provides a semantic segmentation method based on multi-source heterogeneous remote sensing images, comprising the following steps:
[0046]Step 1. Preprocess the training pictures in the existing public remote sensing image data set: the remote sensing image data set is a multi-source heterogeneous data set, which can have different spatial resolutions, have multi-level category labels, and be taken by different satellites , such as: NAIC-2020, GID-15, DeepGlobe or City-OSM, etc.; data enhancement for training pictures: (1) random scaling of pictures according to the ratio of 0.7-1.3; (2) random horizontal flipping of training pictures and random Flip vertically; (3) randomly crop 256×256 samples from the picture; (4) normalize the picture using channel mean and standard deviation.
[0047] Step 2. Build a multi-level segmentation ...
PUM
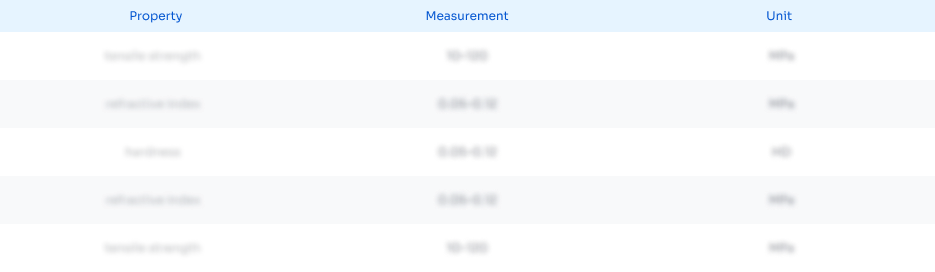
Abstract
Description
Claims
Application Information
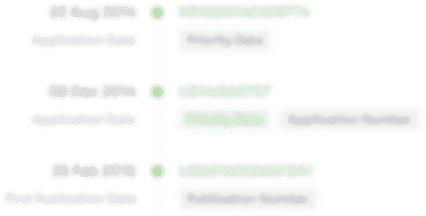
- R&D Engineer
- R&D Manager
- IP Professional
- Industry Leading Data Capabilities
- Powerful AI technology
- Patent DNA Extraction
Browse by: Latest US Patents, China's latest patents, Technical Efficacy Thesaurus, Application Domain, Technology Topic.
© 2024 PatSnap. All rights reserved.Legal|Privacy policy|Modern Slavery Act Transparency Statement|Sitemap