Speech emotion recognition method and system based on semi-supervised adversarial variation self-coding
A speech emotion recognition and semi-supervised technology, applied in speech recognition, speech analysis, instruments, etc., can solve problems such as the quality of emotional feature representation needs to be improved, performance input data disturbance, weak generalization ability, etc., to improve accuracy and generalization ability, the ability to improve feature distribution, and the effect of improving feature representation quality
- Summary
- Abstract
- Description
- Claims
- Application Information
AI Technical Summary
Problems solved by technology
Method used
Image
Examples
Embodiment Construction
[0051] The present invention will be further described below in conjunction with the accompanying drawings and specific preferred embodiments, but the protection scope of the present invention is not limited thereby.
[0052] Such as figure 1 As shown, the steps of the speech emotion recognition method based on semi-supervised adversarial variational self-encoding in this embodiment include:
[0053] S1. SSAVAE model construction: build a generative confrontation network GAN and combine the semi-supervised variational autoencoder model SSVAE and the generative confrontation network to construct a speech emotion recognition model, in which the input data with emotional label data and the corresponding emotional label are used as input, Make the generated hidden layer features conform to the distribution characteristics of emotional labels, and treat the data without emotional labels in the input data as the missing type of emotional label attributes, that is, use the emotional ...
PUM
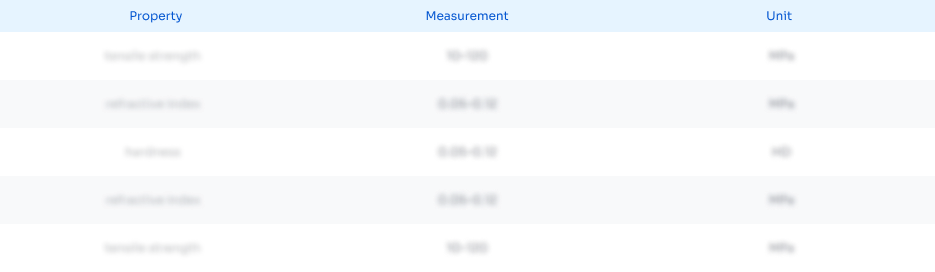
Abstract
Description
Claims
Application Information
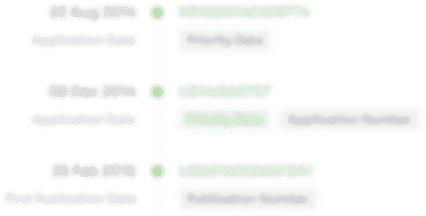
- R&D Engineer
- R&D Manager
- IP Professional
- Industry Leading Data Capabilities
- Powerful AI technology
- Patent DNA Extraction
Browse by: Latest US Patents, China's latest patents, Technical Efficacy Thesaurus, Application Domain, Technology Topic, Popular Technical Reports.
© 2024 PatSnap. All rights reserved.Legal|Privacy policy|Modern Slavery Act Transparency Statement|Sitemap|About US| Contact US: help@patsnap.com