Fault diagnosis method of equipment analog circuit based on probabilistic neural network
A probabilistic neural network, a technology for simulating circuit faults, applied in biological neural network models, neural architectures, electrical and digital data processing, etc. Effect
- Summary
- Abstract
- Description
- Claims
- Application Information
AI Technical Summary
Problems solved by technology
Method used
Image
Examples
Embodiment 1
[0144] In this example specifying ψ 1 、ψ 2 The role of the two parameters, with image 3 The circuit shown is the research object, the selected state set is shown in Table 1 below, and the fault feature at the output node OUT is selected as the BCM value. Since there is only one output feature—the BCM value of the voltage at the OUT node, the sensitivity matrix becomes a 1×11-dimensional row vector Each element represents the sensitivity of the corresponding component out-of-tolerance condition when the fault feature is BCM value. The measured values are shown in Table 2 below.
[0145] Table 1 Circuit state set
[0146]
[0147] Table 2 Sensitivity of measured values with BCM value as fault feature
[0148]
[0149] Combining Table 1 and Table 2, it can be seen that:
[0150] (1) Except state X 2 、X 3 、X 4 、X 5 、X 6 、X 7 In addition, the sensitivities are too small, if the first type of threshold parameter ψ 1 =1%, then the remaining states with too lo...
Embodiment 2
[0158] In this embodiment, the diagnosis effect of the fault diagnosis method of the present invention is verified. Still use the attached image 3 In the circuit diagram in the circuit, the fault state of the components in the circuit is set as positive (↑) and negative (↓) 20% of the nominal value, and the node OUT is the output test point. The simulation platform is Orcad CIS 7.0 and Matlab 8.5, and the number of sampling points is 1024. Take the circuit state set as shown in the part of the circuit state in Table 3 below.
[0159] Table 3 Partial circuit status
[0160]
[0161] When the input excitation signal is set as v=10sin(ωt), the BCM value is calculated for different fault states of the circuit. Furthermore, 100 sets of data in each state of ω=100 are taken, among which 40 sets are used as training samples and 60 sets are used as test samples. Utilize the algorithm setting Z=80% among the present invention, obtain parameter σ=0.8638, ψ 1 =0.813%, ψ 2 =0.09...
Embodiment 3
[0167] to attach Figure 5 Take part of the circuit in a certain type of missile as an example to verify the effectiveness of the algorithm in the present invention. Assume that the fault has been located to the circuit part shown in the figure, but the specific location and parameters are unknown. Through the parameter optimization method in the present invention, the variable resistor RP3 has low sensitivity to the output characteristics at the test point OUT, so it is eliminated, and the remaining measurable circuit faults are sorted into Table 5, together with the normal state, there are 19 categories in total.
[0168] Table 5 The measurable fault state table of the active filter circuit of a certain missile
[0169]
[0170] Adjust the corresponding components in the circuit according to the fault values of each component shown in Table 5, collect signals at OUT and process the samples using the SVD-LCD-BCM algorithm in the present invention, and input the processe...
PUM
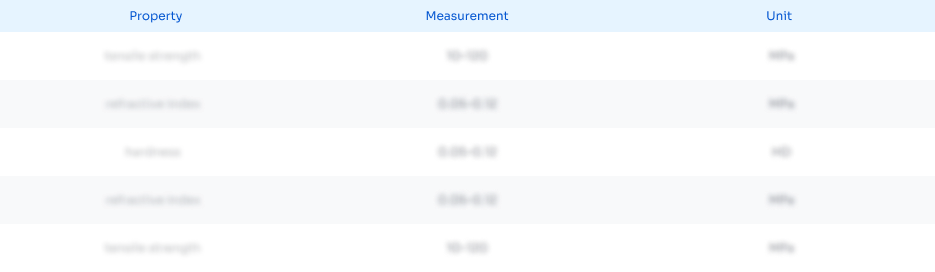
Abstract
Description
Claims
Application Information
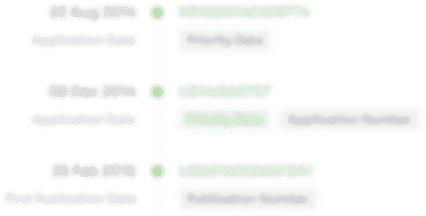
- R&D Engineer
- R&D Manager
- IP Professional
- Industry Leading Data Capabilities
- Powerful AI technology
- Patent DNA Extraction
Browse by: Latest US Patents, China's latest patents, Technical Efficacy Thesaurus, Application Domain, Technology Topic, Popular Technical Reports.
© 2024 PatSnap. All rights reserved.Legal|Privacy policy|Modern Slavery Act Transparency Statement|Sitemap|About US| Contact US: help@patsnap.com