Semi-supervised graph representation learning method based on fusion of transfer learning and deep learning and device thereof
A deep learning and transfer learning technology, applied in the information field, can solve the problems of low quality of graph representation, poor scalability, and a large number of other problems, so as to save computing time, simplify the training process, and save computing resources.
- Summary
- Abstract
- Description
- Claims
- Application Information
AI Technical Summary
Problems solved by technology
Method used
Image
Examples
Embodiment Construction
[0026] In order to make the above objects, features and advantages of the present invention more comprehensible, the present invention will be further described in detail below through specific embodiments and accompanying drawings.
[0027] Main content and purpose of the present invention include:
[0028] 1) Through a semi-supervised graph representation learning method, capture the general structural information in the unlabeled graph data to provide useful representation information or parameters for downstream target tasks and use the labeled data to fine-tune the model to solve the problem of labels in the original data. Fewer problems, save the cost of manual labeling, and effectively combine unlabeled data and labeled data to improve the generalization performance of the model and improve the performance of downstream target tasks.
[0029] 2) Through a pre-training mechanism, two different levels of sub-tasks are designed to learn the general representation of the in...
PUM
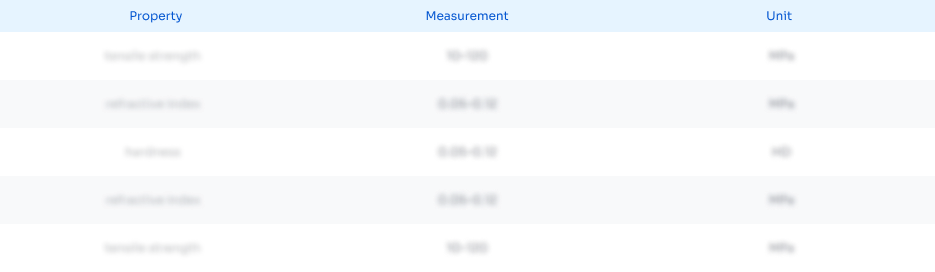
Abstract
Description
Claims
Application Information
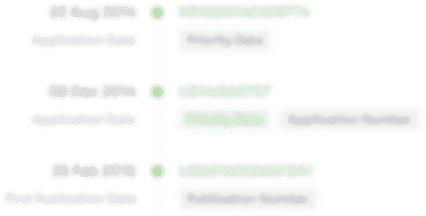
- R&D Engineer
- R&D Manager
- IP Professional
- Industry Leading Data Capabilities
- Powerful AI technology
- Patent DNA Extraction
Browse by: Latest US Patents, China's latest patents, Technical Efficacy Thesaurus, Application Domain, Technology Topic, Popular Technical Reports.
© 2024 PatSnap. All rights reserved.Legal|Privacy policy|Modern Slavery Act Transparency Statement|Sitemap|About US| Contact US: help@patsnap.com