Residual error and batch normalization-based neural network noisy vocal print identification method
A neural network and recognition method technology, applied in the field of voiceprint recognition, can solve problems such as network gradient disappearance, poor robustness, degradation, etc., to improve the ability of network generalization, reduce information loss, and voiceprint recognition rate Improved effect
- Summary
- Abstract
- Description
- Claims
- Application Information
AI Technical Summary
Problems solved by technology
Method used
Image
Examples
Embodiment Construction
[0014] Below in conjunction with the accompanying drawings and preferred embodiments, the specific implementation, structure, features and efficacy of a neural network noise pattern recognition method based on residual and batch normalization proposed according to the present invention will be described in detail as follows .
[0015] see figure 1 , a kind of neural network noise pattern recognition method based on residual error and batch normalization of the present invention, comprises the following steps:
[0016] (1) Data preparation: Randomly add reverberation, noise, and music into the data set to obtain a data set in a noisy environment, and perform data preprocessing, noise addition, mute deletion, and less than The process of 5s speech, extracts corresponding Mel cepstral coefficient respectively to described speech signal;
[0017] (2) Build a ResTDNN network:
[0018] Modify the input layer of the residual neural network so that it can correct the output layer. ...
PUM
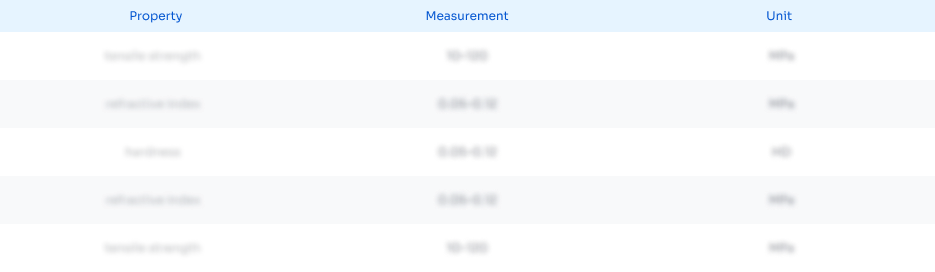
Abstract
Description
Claims
Application Information
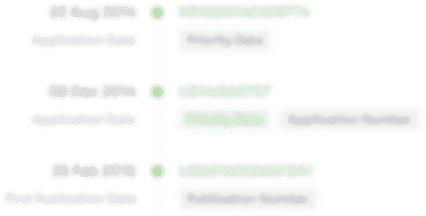
- Generate Ideas
- Intellectual Property
- Life Sciences
- Materials
- Tech Scout
- Unparalleled Data Quality
- Higher Quality Content
- 60% Fewer Hallucinations
Browse by: Latest US Patents, China's latest patents, Technical Efficacy Thesaurus, Application Domain, Technology Topic, Popular Technical Reports.
© 2025 PatSnap. All rights reserved.Legal|Privacy policy|Modern Slavery Act Transparency Statement|Sitemap|About US| Contact US: help@patsnap.com