Steel and iron material fatigue performance prediction method based on transfer learning guided by mechanical theory
A steel material, transfer learning technology, applied in electrical digital data processing, instruments, computer-aided design and other directions, can solve the problem of high cost attribute prediction of materials, and achieve the effect of improving the research and development rate and reducing the data volume requirements.
- Summary
- Abstract
- Description
- Claims
- Application Information
AI Technical Summary
Problems solved by technology
Method used
Image
Examples
Embodiment Construction
[0042] The specific implementation manners of the present invention will be further described in detail below in conjunction with the accompanying drawings and embodiments. The following examples are used to illustrate the present invention, but are not intended to limit the scope of the present invention.
[0043] A method for predicting fatigue performance of steel materials based on transfer learning guided by mechanical theory, such as figure 1 shown, including the following steps:
[0044] Step 1: Establish a data set of iron and steel material composition, process and performance; this embodiment collects the data of the target material from a large number of documents, and establishes a source data set of no less than 400 pieces of data and a target data set of about 100 pieces of data
[0045] Step 1.1: Obtain the composition, process and corresponding source performance of m materials in iron and steel materials. The composition, process and source performance of eac...
PUM
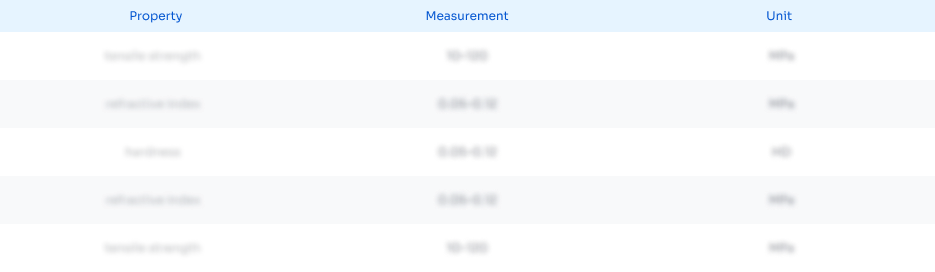
Abstract
Description
Claims
Application Information
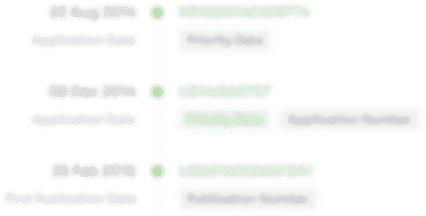
- Generate Ideas
- Intellectual Property
- Life Sciences
- Materials
- Tech Scout
- Unparalleled Data Quality
- Higher Quality Content
- 60% Fewer Hallucinations
Browse by: Latest US Patents, China's latest patents, Technical Efficacy Thesaurus, Application Domain, Technology Topic, Popular Technical Reports.
© 2025 PatSnap. All rights reserved.Legal|Privacy policy|Modern Slavery Act Transparency Statement|Sitemap|About US| Contact US: help@patsnap.com