Robot confrontation method based on evolutionary reinforcement learning
A reinforcement learning and robotics technology, applied in neural learning methods, instruments, artificial life, etc., can solve problems such as poor exploration ability, difficult continuous control of robots, complex modeling process, etc., to achieve the effect of improving exploration ability and stability
- Summary
- Abstract
- Description
- Claims
- Application Information
AI Technical Summary
Problems solved by technology
Method used
Image
Examples
Embodiment Construction
[0069] Exemplary embodiments of the present disclosure will be described in more detail below with reference to the accompanying drawings. Although exemplary embodiments of the present disclosure are shown in the drawings, it should be understood that the present disclosure may be embodied in various forms and should not be limited by the embodiments set forth herein. Rather, these embodiments are provided for more thorough understanding of the present disclosure and to fully convey the scope of the present disclosure to those skilled in the art.
[0070] The present invention uses the evolutionary reinforcement learning method, which is a combination of deep reinforcement learning and evolutionary thinking. The deep reinforcement learning method does not require modeling, and can realize end-to-end control of the robot, and has a certain generalization ability, which can effectively solve the current problems. There are problems with the method, and evolutionary thinking uses...
PUM
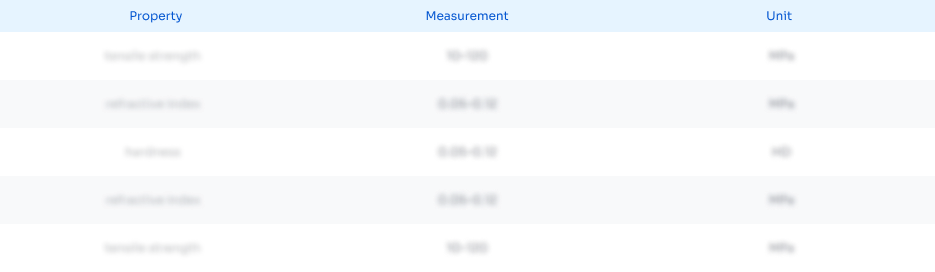
Abstract
Description
Claims
Application Information
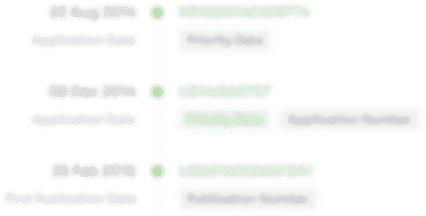
- R&D Engineer
- R&D Manager
- IP Professional
- Industry Leading Data Capabilities
- Powerful AI technology
- Patent DNA Extraction
Browse by: Latest US Patents, China's latest patents, Technical Efficacy Thesaurus, Application Domain, Technology Topic, Popular Technical Reports.
© 2024 PatSnap. All rights reserved.Legal|Privacy policy|Modern Slavery Act Transparency Statement|Sitemap|About US| Contact US: help@patsnap.com