D2D communication network slice allocation method based on deep reinforcement learning
A technology of reinforcement learning and communication network, applied in the field of D2D communication network slice allocation based on deep reinforcement learning, can solve the problem that the agent cannot obtain the strategy, achieve the effect of improving efficiency, optimizing experience quality, and meeting communication requirements
- Summary
- Abstract
- Description
- Claims
- Application Information
AI Technical Summary
Problems solved by technology
Method used
Image
Examples
Embodiment 1
[0083] This embodiment provides a D2D communication network slice allocation method based on deep reinforcement learning, such as figure 1 shown, including the following steps:
[0084] S1: Classify communication services according to service types, and establish resource allocation models for multi-service slices and D2D slices;
[0085] S2: Construct a reinforcement learning model for slice resource allocation based on the Dueling DDQN algorithm;
[0086] S3: Define the current state s of the business slice, the state s′ at the next moment, the current action a, and the reward r of the system constructed by the state and action for the agent in the Dueling DDQN algorithm;
[0087] S4: Use experience playback to learn Dueling DDQN, and finally get the optimal solution for slice resource allocation.
[0088] In step S1, the communication services are classified according to service types, specifically, they are divided into control, data collection, media and D2D communicati...
PUM
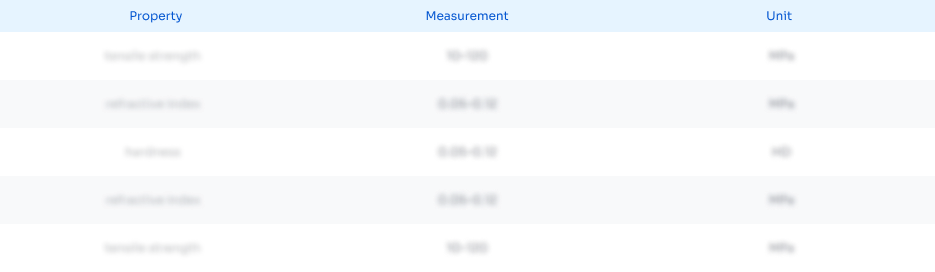
Abstract
Description
Claims
Application Information
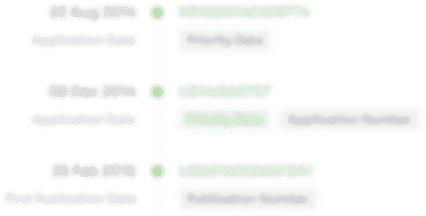
- R&D
- Intellectual Property
- Life Sciences
- Materials
- Tech Scout
- Unparalleled Data Quality
- Higher Quality Content
- 60% Fewer Hallucinations
Browse by: Latest US Patents, China's latest patents, Technical Efficacy Thesaurus, Application Domain, Technology Topic, Popular Technical Reports.
© 2025 PatSnap. All rights reserved.Legal|Privacy policy|Modern Slavery Act Transparency Statement|Sitemap|About US| Contact US: help@patsnap.com