DBT lump automatic segmentation method based on expansion depth convolutional neural network
A neural network and deep convolution technology, which is applied in the field of DBT mass segmentation based on dilated deep convolutional neural network, can solve the problems of lack of a unified standard for selection, time-consuming, and large amount of calculation, so as to suppress the category imbalance. effect, improve accuracy and robustness, improve generalization ability
- Summary
- Abstract
- Description
- Claims
- Application Information
AI Technical Summary
Problems solved by technology
Method used
Image
Examples
Embodiment 1
[0039] Embodiment 1, the DBT mass automatic segmentation method based on expansion depth convolutional neural network, such as Figure 1-2 As shown, the DBT image is segmented to obtain sliced images, and each sliced image is preprocessed to extract image blocks, and then the central voxels of all image blocks are classified through the trained expanded deep convolutional neural network segmentation model. Determine that the category of the central voxel is the breast normal tissue area (C0) or the breast mass area (C1), thereby completing the segmentation of the DBT body image, and then the result obtained through post-processing is the final DBT mass segmentation result; The expanded deep convolutional neural network segmentation model is hereinafter referred to as the segmentation model, and the automatic segmentation method for DBT tumors based on the segmentation model includes the following steps:
[0040] S01. Collect images
[0041]The digital breast three-dimensi...
PUM
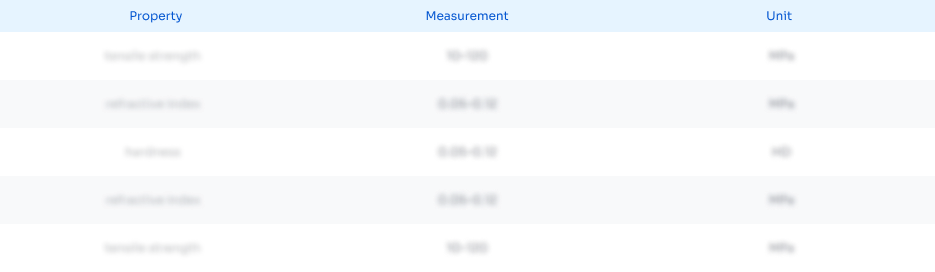
Abstract
Description
Claims
Application Information
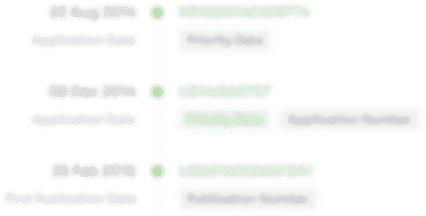
- R&D Engineer
- R&D Manager
- IP Professional
- Industry Leading Data Capabilities
- Powerful AI technology
- Patent DNA Extraction
Browse by: Latest US Patents, China's latest patents, Technical Efficacy Thesaurus, Application Domain, Technology Topic.
© 2024 PatSnap. All rights reserved.Legal|Privacy policy|Modern Slavery Act Transparency Statement|Sitemap