Human body behavior recognition method based on multi-scale attention map convolutional network
A convolutional network and recognition method technology, applied in the field of human behavior recognition based on multi-scale attention graph convolutional network, can solve the problem that the feature information is difficult to accurately express human behavior, joint feature redundancy, and the accuracy of human behavior recognition is not good, etc. To improve the recognition accuracy, ensure the recognition effect, and improve the recognition efficiency
- Summary
- Abstract
- Description
- Claims
- Application Information
AI Technical Summary
Problems solved by technology
Method used
Image
Examples
Embodiment
[0049] The applicant found in actual research that the graph convolutional network explores the spatio-temporal dependencies between human joints by constructing a 3D skeleton spatio-temporal graph of the human body. However, the skeleton graph structure is predefined, which cannot represent the changeable human body under different behaviors. At the same time, the motion information that highly expresses specific behaviors often only needs the spatio-temporal features of a few key nodes. Therefore, there is a problem of redundancy in the joint features in the skeleton sequence, and it is necessary to add varying degrees of attention to determine its importance. In addition, existing human body recognition methods usually use feature vectors that only contain 3D skeleton joint points, which lack multi-scale information that can express skeleton changes and motion changes, and cannot fully express human behavior.
[0050] Based on the above findings, the applicant designed the ...
PUM
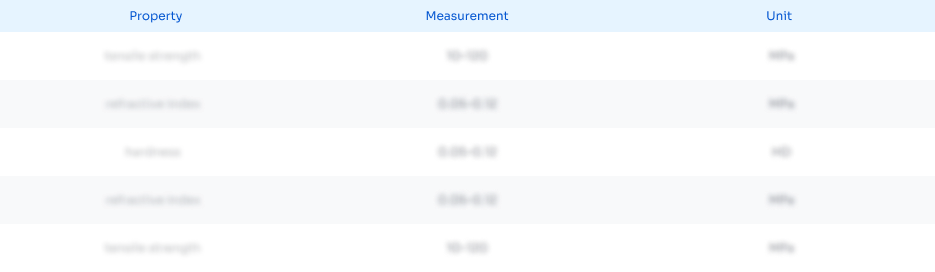
Abstract
Description
Claims
Application Information
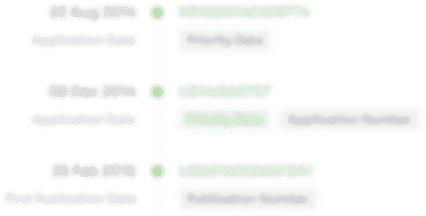
- R&D Engineer
- R&D Manager
- IP Professional
- Industry Leading Data Capabilities
- Powerful AI technology
- Patent DNA Extraction
Browse by: Latest US Patents, China's latest patents, Technical Efficacy Thesaurus, Application Domain, Technology Topic, Popular Technical Reports.
© 2024 PatSnap. All rights reserved.Legal|Privacy policy|Modern Slavery Act Transparency Statement|Sitemap|About US| Contact US: help@patsnap.com