Sample classification method based on active learning and neural network
A neural network and active learning technology, applied in the field of machine learning, can solve the problems of incomplete measurement of uncertainty, low model classification accuracy, etc., to reduce the time and money cost, improve the classification effect, and reduce the number of effects.
- Summary
- Abstract
- Description
- Claims
- Application Information
AI Technical Summary
Problems solved by technology
Method used
Image
Examples
Embodiment Construction
[0020] The present invention will be described in further detail below in conjunction with the accompanying drawings and specific embodiments.
[0021] Taking a three-layer feed-forward neural network model as an example, the active learning sample screening method according to the present invention is described. However, those skilled in the art should understand that the present invention is not limited to this neural network, but can be applied to other multi-classification neural networks whose output uses Softmax.
[0022] figure 1 The three-layer feed-forward neural network model shown is a model for multi-sample classification tasks. Taking sample x as an example, the input layer is the 0th layer, which represents the feature vector of sample x, the middle two layers are hidden layers, and the output The number n of layer nodes represents the number of labels that sample x may take, w (i) Represents the connection weight matrix between the i-1th layer and the i-th lay...
PUM
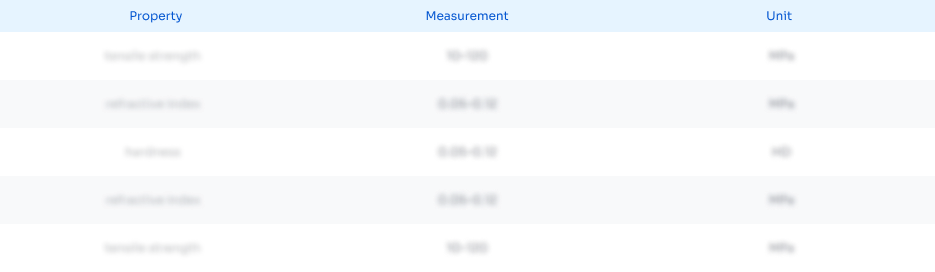
Abstract
Description
Claims
Application Information
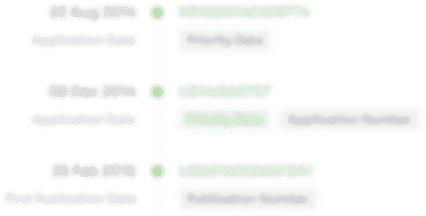
- R&D Engineer
- R&D Manager
- IP Professional
- Industry Leading Data Capabilities
- Powerful AI technology
- Patent DNA Extraction
Browse by: Latest US Patents, China's latest patents, Technical Efficacy Thesaurus, Application Domain, Technology Topic, Popular Technical Reports.
© 2024 PatSnap. All rights reserved.Legal|Privacy policy|Modern Slavery Act Transparency Statement|Sitemap|About US| Contact US: help@patsnap.com