Deep learning SAR image ship identification method based on self-supervision condition
A deep learning and recognition method technology, applied in the field of SAR image ship target detection, can solve the problems of false detection results, incomplete detection results, and inability to achieve rapid recognition of radar targets.
- Summary
- Abstract
- Description
- Claims
- Application Information
AI Technical Summary
Problems solved by technology
Method used
Image
Examples
Embodiment Construction
[0047] The present invention provides a deep learning SAR image ship recognition method based on self-supervised conditions. First, the SAR data is preprocessed, and the image pixel threshold is obtained by using the cumulative inverse exponential probability distribution, and the threshold is used to perform rapid segmentation to obtain a binary image. Then perform eight-neighborhood connection processing on the binarized image to obtain the geometric information of the candidate target, construct a SAR ship slice data set according to the geometric information of the candidate target, establish a CNN network model, and train and optimize it for use in Self-supervised identification of ship targets. The technical solution of the present invention will be further described below in conjunction with the accompanying drawings and embodiments.
[0048] like figure 1 As shown, the process of the embodiment of the present invention includes the following steps:
[0049] Step 1, c...
PUM
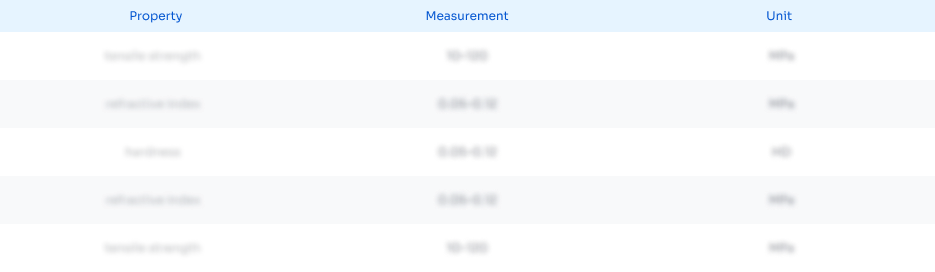
Abstract
Description
Claims
Application Information
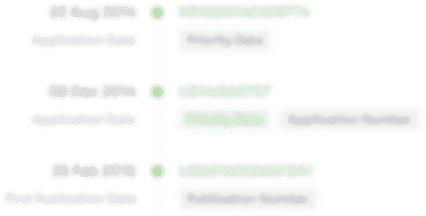
- R&D Engineer
- R&D Manager
- IP Professional
- Industry Leading Data Capabilities
- Powerful AI technology
- Patent DNA Extraction
Browse by: Latest US Patents, China's latest patents, Technical Efficacy Thesaurus, Application Domain, Technology Topic, Popular Technical Reports.
© 2024 PatSnap. All rights reserved.Legal|Privacy policy|Modern Slavery Act Transparency Statement|Sitemap|About US| Contact US: help@patsnap.com