Small sample target detection method based on attention and contrast learning
A technology of target detection and attention, applied in the field of image processing and artificial intelligence, can solve problems such as insufficient utilization, different parameter settings, knowledge transfer and forgetting, etc.
- Summary
- Abstract
- Description
- Claims
- Application Information
AI Technical Summary
Problems solved by technology
Method used
Image
Examples
Embodiment 1
[0066] Embodiment 1: as Figure 1-6 As shown, a small-sample object detection method based on attention and contrastive learning is characterized in that it includes a small-sample mosaic data enhancement module, a coordinate compensation attention region proposal network, a new and old class discrimination module, and a comparative learning module.
[0067] Small sample mosaic data enhancement module, including sample selection strategy and mosaic data enhancement method;
[0068] Coordinate compensation attention region proposal network, including region proposal network, regression coordinate compensation attention branch, coordinate compensation loss;
[0069] New and old class discrimination module, including feature extraction layer and class discrimination layer;
[0070] Contrastive learning module, including feature map layer, contrastive loss and joint training strategy;
[0071] On the basis of the general two-stage object detection baseline R-CNN, the above modul...
Embodiment 2
[0122] Embodiment 2: This embodiment describes the present invention in combination with specific data.
[0123] The model training is divided into two stages: the first stage is base class training; the second stage is the fine-tuning stage for new classes with small samples. Among them, the number of training samples of the base class is sufficient, and the training data of the new class is divided into 1, 3, 5 and 10 samples for each class according to the task. The model is trained and verified on the COCO dataset and the VOC dataset respectively. The COCO dataset has 80 categories, of which 60 are base categories and 20 are new categories; the VOC dataset has 20 categories, of which 15 1 class is the base class and 5 classes are new classes.
[0124] Hyperparameter settings: Gradient descent uses standard SGD, momentum is 0.9, weight decay is e -4 , batchsize is 16. The software environment is Ubuntu18.04, Cuda10.2, Pytorch1.4.0, Python3.6; the hardware environment is 8...
PUM
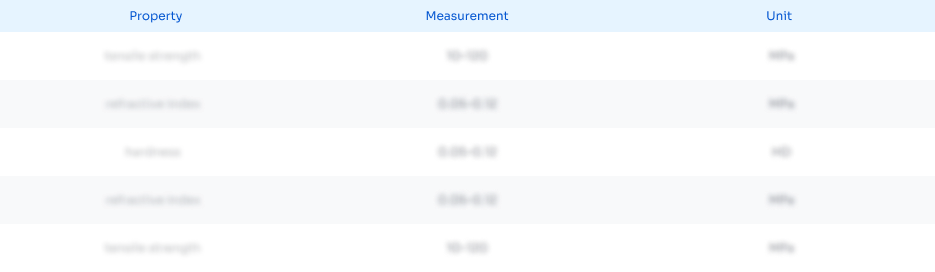
Abstract
Description
Claims
Application Information
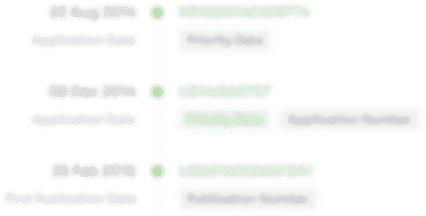
- R&D Engineer
- R&D Manager
- IP Professional
- Industry Leading Data Capabilities
- Powerful AI technology
- Patent DNA Extraction
Browse by: Latest US Patents, China's latest patents, Technical Efficacy Thesaurus, Application Domain, Technology Topic, Popular Technical Reports.
© 2024 PatSnap. All rights reserved.Legal|Privacy policy|Modern Slavery Act Transparency Statement|Sitemap|About US| Contact US: help@patsnap.com