Deep learning rolling bearing fault diagnosis method based on feature fusion and hybrid enhancement
A rolling bearing and fault diagnosis technology, applied in the field of deep learning, can solve problems such as relationships that have not been modeled, achieve good domain adaptability, improve domain adaptability, and enhance generalization capabilities
- Summary
- Abstract
- Description
- Claims
- Application Information
AI Technical Summary
Problems solved by technology
Method used
Image
Examples
Embodiment 1
[0033] see Figure 1-Figure 3 , this embodiment 1 provides a method for deep learning rolling bearing fault diagnosis based on feature fusion and mixed class enhancement, the method includes the following steps:
[0034] Step S1, extracting the time domain features, frequency domain features, working condition features and time difference features in the original one-dimensional vibration signal, and combining with the original one-dimensional vibration signal to form a new one-dimensional signal sample;
[0035] Specifically, in this embodiment, the original one-dimensional vibration signal uses the experimental data of the bearing in the laboratory of Case Western Reserve University in the United States, and the experimental data adopts the data of the driving end bearing at a sampling frequency of 12K and is obtained by an accelerometer. In this experimental data, there are four types of faults in rolling bearings: inner ring faults, rolling element faults, outer ring fault...
PUM
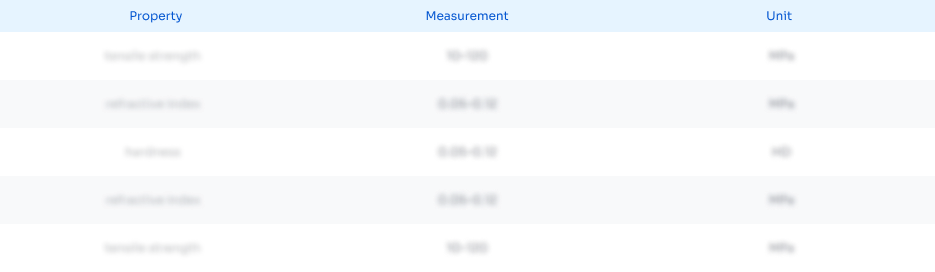
Abstract
Description
Claims
Application Information
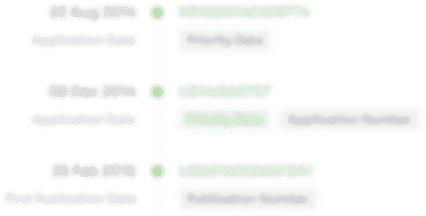
- R&D Engineer
- R&D Manager
- IP Professional
- Industry Leading Data Capabilities
- Powerful AI technology
- Patent DNA Extraction
Browse by: Latest US Patents, China's latest patents, Technical Efficacy Thesaurus, Application Domain, Technology Topic.
© 2024 PatSnap. All rights reserved.Legal|Privacy policy|Modern Slavery Act Transparency Statement|Sitemap