Atmospheric pollutant prediction method and system based on deep learning model
An air pollutant and deep learning technology, applied in the field of air pollutant prediction based on deep learning model, can solve the problems of neglect, lack of complex underlying surface information of sites, failure to effectively use multi-source data, etc., to improve the prediction ability Effect
- Summary
- Abstract
- Description
- Claims
- Application Information
AI Technical Summary
Problems solved by technology
Method used
Image
Examples
Embodiment Construction
[0067] The following will clearly and completely describe the technical solutions in the embodiments of the present invention with reference to the accompanying drawings in the embodiments of the present invention. Obviously, the described embodiments are only some, not all, embodiments of the present invention. Based on the embodiments of the present invention, all other embodiments obtained by persons of ordinary skill in the art without creative efforts fall within the protection scope of the present invention.
[0068] figure 1 Shown is a flow chart of an air pollutant prediction method based on a deep learning model provided by an embodiment of the present invention; as figure 1 As shown, the air pollutant prediction method based on deep learning model provided by the present invention comprises the following steps:
[0069] Step S1, converting the unstructured input data based on the monitoring site into graph-structured data containing structural information.
[0070]...
PUM
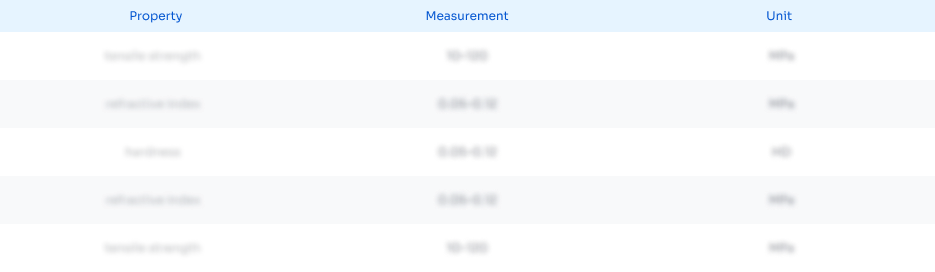
Abstract
Description
Claims
Application Information
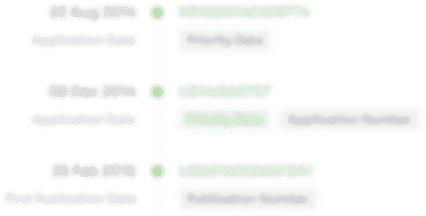
- R&D
- Intellectual Property
- Life Sciences
- Materials
- Tech Scout
- Unparalleled Data Quality
- Higher Quality Content
- 60% Fewer Hallucinations
Browse by: Latest US Patents, China's latest patents, Technical Efficacy Thesaurus, Application Domain, Technology Topic, Popular Technical Reports.
© 2025 PatSnap. All rights reserved.Legal|Privacy policy|Modern Slavery Act Transparency Statement|Sitemap|About US| Contact US: help@patsnap.com