Student performance prediction method based on hybrid deep learning and attention mechanism
A technology of deep learning and prediction methods, applied in neural learning methods, prediction, biological neural network models, etc., can solve problems such as large number of parameters, lack of feature extraction process, model overfitting, etc., to improve prediction accuracy, improve Training efficiency, reducing the effect of overfitting
- Summary
- Abstract
- Description
- Claims
- Application Information
AI Technical Summary
Problems solved by technology
Method used
Image
Examples
Embodiment Construction
[0025] In order to make the purpose, technical solution and advantages of the present invention clearer, the present invention will be further elaborated below in combination with specific examples and with reference to the accompanying drawings.
[0026] The present invention describes the specific implementation process of the method of the present invention by taking student performance prediction based on hybrid deep learning and attention mechanism as an example.
[0027] The model framework of the present invention is as figure 1 shown.
[0028] The overall process of the present invention is as figure 2 shown. Combined with the schematic diagram to illustrate the specific steps:
[0029] Step 1. Download the Open University Learning Analysis Dataset (OULAD) from the official website, filter and preprocess the data.
[0030] Step 2. Since there are many types of student learning activities in OULAD, however, different courses focus on different types of learning act...
PUM
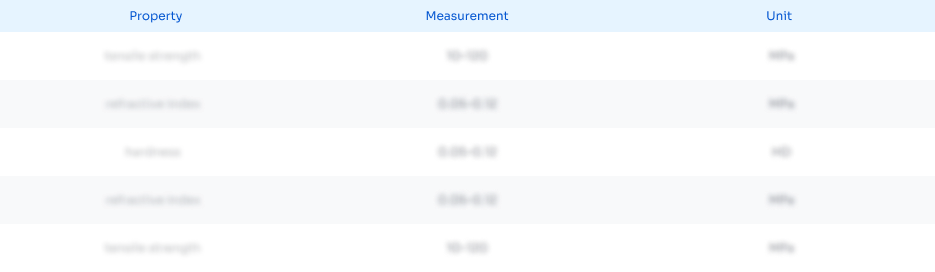
Abstract
Description
Claims
Application Information
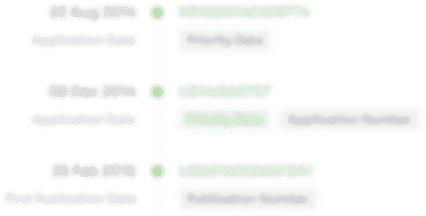
- R&D Engineer
- R&D Manager
- IP Professional
- Industry Leading Data Capabilities
- Powerful AI technology
- Patent DNA Extraction
Browse by: Latest US Patents, China's latest patents, Technical Efficacy Thesaurus, Application Domain, Technology Topic, Popular Technical Reports.
© 2024 PatSnap. All rights reserved.Legal|Privacy policy|Modern Slavery Act Transparency Statement|Sitemap|About US| Contact US: help@patsnap.com