Super-resolution model compression and acceleration method based on self-distillation contrast learning
A super-resolution and resolution technology, applied in the field of super-resolution, can solve the problems of increased memory and calculation amount, large amount of super-resolution model parameters and calculation amount, and difficult deployment of super-resolution model, so as to reduce the amount of parameters. and computational complexity, the effect of strong realism
- Summary
- Abstract
- Description
- Claims
- Application Information
AI Technical Summary
Problems solved by technology
Method used
Image
Examples
Embodiment 1
[0041] See attached figure 1 , in the training stage, the present invention is mainly divided into three parts: data set preprocessing, teacher network (teacher super-resolution model) pre-training and student network (student super-resolution model) training.
[0042] A1: The data sets used are public data sets DIV2K, Set5 and Urban100. DIV2K contains 1000 pictures with a resolution of 2K, 800 of which are selected as the training set, and the remaining 200 constitute the test set. The verification set consists of DIV2K, Set5, and Urban100 composition. All images use bicubic interpolation to generate corresponding low-resolution images.
[0043] A2: During the training process, all training set images are preprocessed to increase the generalization ability of the model, mainly including random cropping of subimages with a size of 192, horizontal and vertical flipping and other techniques.
[0044] See attached figure 2 , the training and loss function calculation process ...
PUM
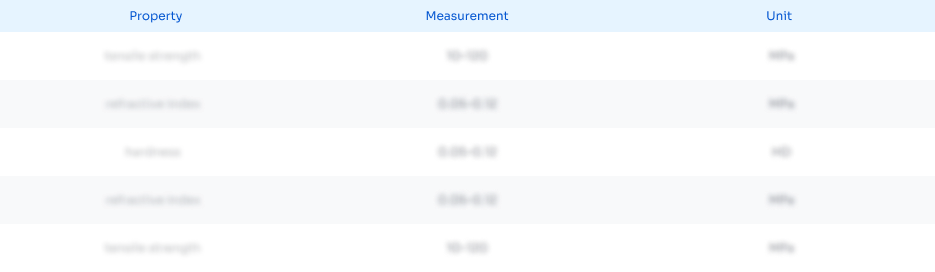
Abstract
Description
Claims
Application Information
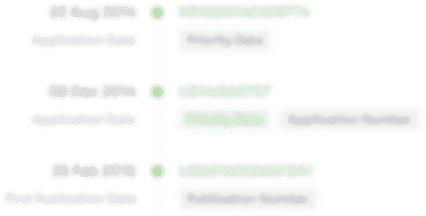
- R&D Engineer
- R&D Manager
- IP Professional
- Industry Leading Data Capabilities
- Powerful AI technology
- Patent DNA Extraction
Browse by: Latest US Patents, China's latest patents, Technical Efficacy Thesaurus, Application Domain, Technology Topic, Popular Technical Reports.
© 2024 PatSnap. All rights reserved.Legal|Privacy policy|Modern Slavery Act Transparency Statement|Sitemap|About US| Contact US: help@patsnap.com