Deep learning image segmentation method and system based on edge feature extraction
A deep learning and image segmentation technology, which is applied in image analysis, image enhancement, image data processing, etc., can solve the problems of difficult application, low portability, and increased network calculation, and achieve the effect of preventing danger and high segmentation accuracy
- Summary
- Abstract
- Description
- Claims
- Application Information
AI Technical Summary
Problems solved by technology
Method used
Image
Examples
Embodiment 1
[0092] Example 1: High Complexity Environment
[0093] In this embodiment, for a high-complexity environment (a visual environment with many objects and complex spatial positional relationships between objects, and a lot of edge information, such as theaters, classrooms, streets, etc.), the high-complexity traffic scene is input to the edge feature-based The extracted deep learning image segmentation system obtains the image segmentation results as shown in 6.
Embodiment 2
[0094] Example 2: Low Complexity Environment
[0095] In this embodiment, aiming at low-complexity environments (visual environments with few objects and simple spatial relationship between objects, and less edge information, such as scenes such as desks, sky, grass, etc.), low-complexity traffic scenes are input into the edge-based feature-based The extracted deep learning image segmentation system obtains image segmentation results such as Figure 7 shown.
Embodiment 3
[0096] Example 3: Common environment
[0097] In this embodiment, aiming at common environments (daily work and life scenes, such as offices, basketball courts, parks, etc.), the traffic scenes under common environments are input to the deep learning image segmentation system based on edge feature extraction, and the image segmentation results are obtained as follows: Figure 8 shown.
PUM
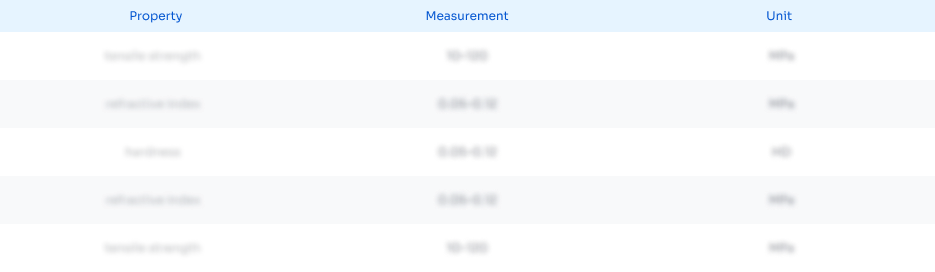
Abstract
Description
Claims
Application Information
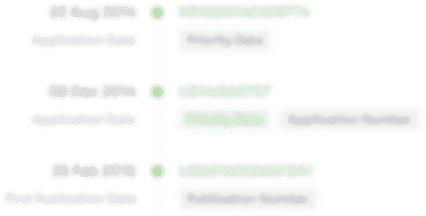
- R&D Engineer
- R&D Manager
- IP Professional
- Industry Leading Data Capabilities
- Powerful AI technology
- Patent DNA Extraction
Browse by: Latest US Patents, China's latest patents, Technical Efficacy Thesaurus, Application Domain, Technology Topic.
© 2024 PatSnap. All rights reserved.Legal|Privacy policy|Modern Slavery Act Transparency Statement|Sitemap