A parameter selection optimization method, system and equipment in random forest model training
A technology of random forest model and parameter optimization algorithm, which is applied in the optimization field of parameter selection in random forest model training, can solve the problems of a balance between accuracy and calculation cost, different efficiency, and inaccurate classification of minority classes, etc., to achieve a good overall situation Effects of improved search capability and classification performance
- Summary
- Abstract
- Description
- Claims
- Application Information
AI Technical Summary
Problems solved by technology
Method used
Image
Examples
Embodiment Construction
[0094] In order to make the object, technical solution and advantages of the present invention more clear, the present invention will be further described in detail below in conjunction with the examples. It should be understood that the specific embodiments described here are only used to explain the present invention, not to limit the present invention.
[0095] Aiming at the problems existing in the prior art, the present invention provides an optimization method, system, and equipment for parameter selection in random forest model training. The present invention will be described in detail below in conjunction with the accompanying drawings.
[0096] Such as figure 1 As shown, the optimization method for parameter selection in the random forest model training provided by the embodiment of the present invention includes the following steps:
[0097] S101, determining the parameter influence of the random forest;
[0098] S102, constructing a parameter optimization algorit...
PUM
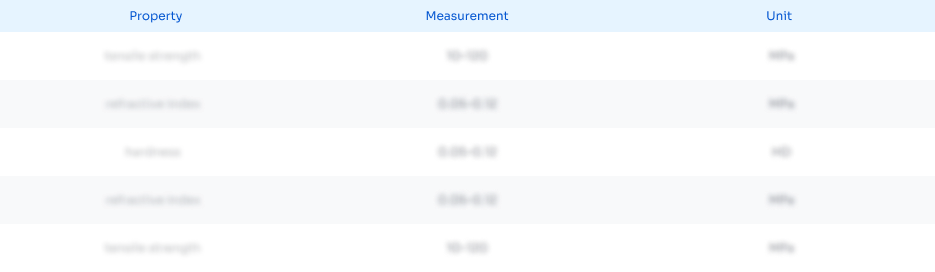
Abstract
Description
Claims
Application Information
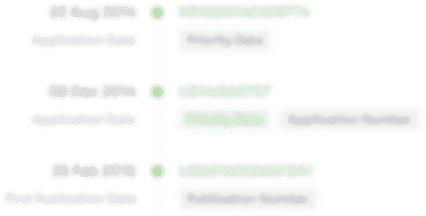
- Generate Ideas
- Intellectual Property
- Life Sciences
- Materials
- Tech Scout
- Unparalleled Data Quality
- Higher Quality Content
- 60% Fewer Hallucinations
Browse by: Latest US Patents, China's latest patents, Technical Efficacy Thesaurus, Application Domain, Technology Topic, Popular Technical Reports.
© 2025 PatSnap. All rights reserved.Legal|Privacy policy|Modern Slavery Act Transparency Statement|Sitemap|About US| Contact US: help@patsnap.com