Random signal identification method based on multilayer neural network and support vector machine
A multi-layer neural network and support vector machine technology, applied in the field of random signal recognition, can solve the problems of complex recognition principle, simple recognition structure, and few recognition methods, and achieve good recognition effect, simple recognition structure, and few recognition methods.
- Summary
- Abstract
- Description
- Claims
- Application Information
AI Technical Summary
Problems solved by technology
Method used
Image
Examples
Embodiment Construction
[0017] The present invention will be described in detail below in conjunction with the accompanying drawings and specific embodiments.
[0018] refer to figure 1 , the identification method of the present invention is based on the frequency-domain random signal principle of a multilayer neural network and a support vector machine, and is implemented according to the following steps:
[0019] Step 1. Establish a finite element model according to the target structure,
[0020] Choose 1D, 2D or 3D elements for modeling according to the actual situation of the target structure. Usually, 2D elements are used for shell structures, 3D elements are used for solid structures, and 1D elements are used for simple rigid connections. The elements are mainly quadrilateral elements and hexahedron elements; element size The empirical value is between 5-30 (for the average unit size, divide the length of the surface edge of the structure by this value to get the number of units on the surface...
PUM
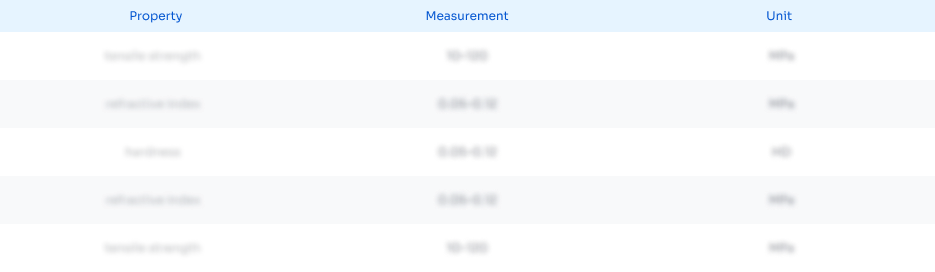
Abstract
Description
Claims
Application Information
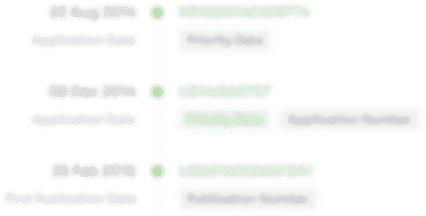
- R&D
- Intellectual Property
- Life Sciences
- Materials
- Tech Scout
- Unparalleled Data Quality
- Higher Quality Content
- 60% Fewer Hallucinations
Browse by: Latest US Patents, China's latest patents, Technical Efficacy Thesaurus, Application Domain, Technology Topic, Popular Technical Reports.
© 2025 PatSnap. All rights reserved.Legal|Privacy policy|Modern Slavery Act Transparency Statement|Sitemap|About US| Contact US: help@patsnap.com