Federated learning system
A learning system and federated technology, applied in the field of artificial intelligence, can solve the problems of low efficiency of federated learning and large amount of data, etc.
- Summary
- Abstract
- Description
- Claims
- Application Information
AI Technical Summary
Problems solved by technology
Method used
Image
Examples
Embodiment 1
[0034] figure 1 It is a training flowchart of a federated learning system provided by the embodiment of the present invention. This embodiment is applicable to the situation of training a federated learning system, especially applicable to the situation of training a vertical federated learning system.
[0035] In this embodiment, the federated learning system includes a coordinating terminal and at least two participating terminals. The participating terminal includes a label holding terminal and at least one feature holding terminal. The coordinating terminal and each participating terminal are connected by communication. Taking the risk control scenario as an example, its characteristic is that the tag is only held by one of the participants (which can be called Guest), while the other participants only have some characteristics of the data (which can be called Host). Through the cooperation of Guest and Host, the effect of the model can be improved, and the purpose of red...
Embodiment 2
[0064] This embodiment provides a preferred embodiment on the basis of the foregoing embodiments. In this embodiment, the tag holder is embodied as a Guest, the feature holder is embodied as a Host, and the collaboration end is embodied as a third-party Coordinator.
[0065] In this embodiment, it is assumed that there are P participating terminals, one of which is a Guest, and the remaining p-1 participating terminals are Hosts, and there is also a third-party Coordinator to coordinate the entire process. Note that the local data of the pth participant after private data alignment is x p , the model parameter is w p . Before iterative training, each participant needs to be initialized. Specifically, each participant generates an initial model parameter w p (0), and then calculate the intermediate value locally, and each Host transmits the ciphertext to the Guest through additive homomorphic encryption (such as Paillier). After the Guest gets all the intermediate values, ...
PUM
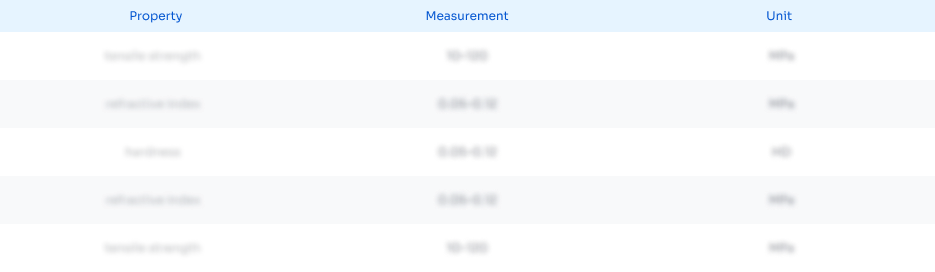
Abstract
Description
Claims
Application Information
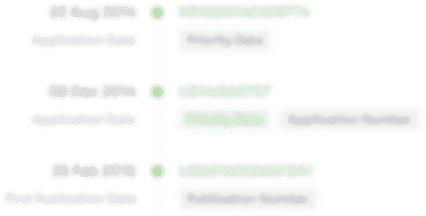
- R&D Engineer
- R&D Manager
- IP Professional
- Industry Leading Data Capabilities
- Powerful AI technology
- Patent DNA Extraction
Browse by: Latest US Patents, China's latest patents, Technical Efficacy Thesaurus, Application Domain, Technology Topic, Popular Technical Reports.
© 2024 PatSnap. All rights reserved.Legal|Privacy policy|Modern Slavery Act Transparency Statement|Sitemap|About US| Contact US: help@patsnap.com