Safe decentralized graph federal learning method
A technology of decentralization and learning methods, applied in the direction of neural learning methods, computer security devices, biological neural network models, etc., can solve problems such as difficult guarantees, time-consuming, protection, etc., to protect data privacy and security, and reduce communication time, the effect of alleviating communication bottlenecks
- Summary
- Abstract
- Description
- Claims
- Application Information
AI Technical Summary
Problems solved by technology
Method used
Image
Examples
Embodiment
[0041] Embodiment: A safe decentralized graph federated learning method of this embodiment, such as figure 1 shown, including the following steps:
[0042] S1: Number all n clients participating in graph federated learning as 1, 2, 3...n in sequence, and one of the clients serves as the training initiator to initialize the parameters of the graph neural network model and the ring communication topology map, and send them to other clients;
[0043] The ring communication topology diagram is matrix A,
[0044] ,
[0045] , , , 1≤i≤n, 1≤j≤n,
[0046] When i=j, A ij ≠0,
[0047] Among them, A ij Indicates the weight coefficient between the client numbered i and the client numbered j, if A ij ≠0 means that the client numbered i can communicate with the client numbered j, if A ij =0 means that the client numbered i cannot communicate with the client numbered j, matrix A is a symmetrical matrix, A ii Indicates the weight coefficient of the client numbered i, Indica...
PUM
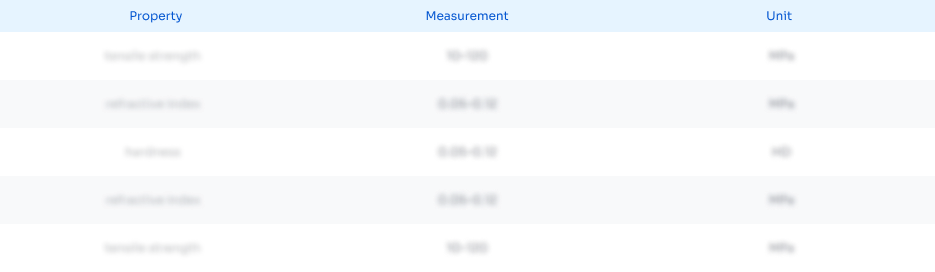
Abstract
Description
Claims
Application Information
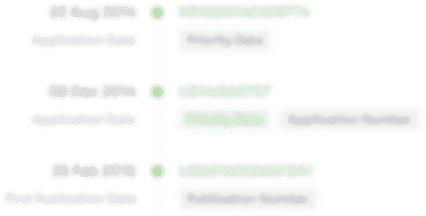
- R&D Engineer
- R&D Manager
- IP Professional
- Industry Leading Data Capabilities
- Powerful AI technology
- Patent DNA Extraction
Browse by: Latest US Patents, China's latest patents, Technical Efficacy Thesaurus, Application Domain, Technology Topic, Popular Technical Reports.
© 2024 PatSnap. All rights reserved.Legal|Privacy policy|Modern Slavery Act Transparency Statement|Sitemap|About US| Contact US: help@patsnap.com